文档简介:
飞桨轻量化推理引擎Paddle Lite
飞桨具有完善的从训练到部署的一系列框架或工具,当读者完成模型的编写和训练后,如果希望将训练好的模型放到手机端或嵌入式端(如摄像头)等去运行,可以使用飞桨轻量化推理引擎Paddle Lite。
Paddle Lite支持包括手机移动端和嵌入式端在内的端侧场景,支持广泛的硬件和平台,是一个高性能、轻量级的深度学习推理引擎。除了和飞桨核心框架无缝对接外,也兼容支持其他训练框架如TensorFlow、Caffe保存的模型(通过X2Paddle工具即可将其他格式的模型转换成飞桨模型)。
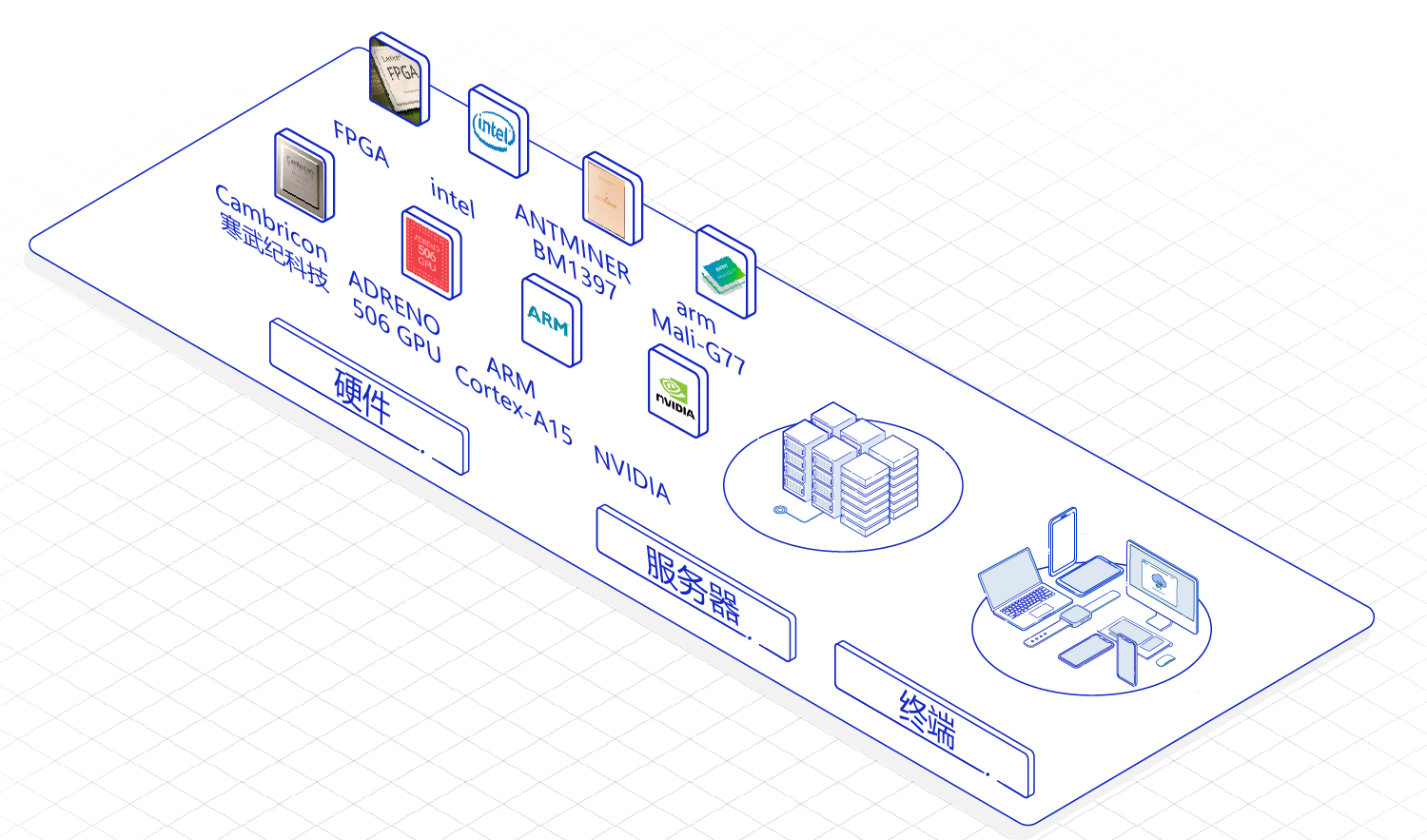
图1 多种推理终端和多种推理硬件层出不穷
随着深度学习的快速发展、特别是小型网络模型的不断成熟,原本应用到云端的深度学习推理,就可以放到终端上来做,比如手机、手表、摄像头、传感器、音响,也就是端智能。此外,可用于深度学习计算的硬件也有井喷之势,从Intel到NVIDIA、ARM、寒武纪等等。相比服务端智能,端智能具有延时低、节省资源、保护数据隐私等优势。目前已经在AI摄像、视觉特效等场景广泛应用。
然而,深度学习推理场景中,多样的平台、不同的芯片对推理库的能力提出了更高的要求。端侧模型的推理经常面临算力和内存的限制,加上日趋异构化的硬件平台和复杂的端侧使用状况,导致端侧推理引擎的架构能力颇受挑战。端侧推理引擎是端智能应用的核心模块,需要在有限算力、有限内存等限制下,高效地利用资源,快速完成推理。因此,飞桨期望提供面向不同业务算法场景、不同训练框架、不同部署环境, 简单、高效、安全的端侧推理引擎。
Paddle Lite的产品特色
为了能够完整地支持众多的硬件架构,实现在这些硬件之上的各种人工智能应用的性能优化,飞桨提供端侧推理引擎Paddle Lite。截止到现在,Paddle Lite已广泛应用于搜索广告、手机百度、百度地图、全民小视频、小度在家等多个重要业务。
Paddle Lite具备如下产品特色:
-
移动端和嵌入端的模型部署工具,可使用其部署飞桨、TensorFlow、Caffe、ONNX等多种平台的主流模型格式,包括MobileNetV1、YOLOv3、UNet、SqueezeNet等主流模型;
-
多种语言的API接口:C++/Java/Python,便于嵌入各种业务程序;
-
丰富的端侧模型:ResNet、EffcientNet、ShuffleNet、MobileNet、Unet、Face Detection、OCR_Attention等;注意因为Lite为了缩小推理库的体积,支持的算子是相对有限的,不像Paddle Inference一样支持Paddle框架的所有算子。但在移动端应用的主流轻量级模型均是支持的,可以放心使用。
-
支持丰富的移动和嵌入端芯片:ARM CPU、Mali GPU、Adreno GPU,昇腾&麒麟NPU,MTK NeuroPilot,RK NPU,MediaTek APU、寒武纪NPU,X86 CPU,NVIDIA GPU,FPGA等多种硬件平台;
-
除了Paddle Lite本身提供的性能优化策略外,还可以结合PaddleSlim可以对模型进行压缩和量化,以达到更好的性能。
Paddle Lite推理部署流程
使用Paddle Lite对模型进行推理部署的流程分两个阶段:
-
模型训练阶段:主要解决模型训练,利用标注数据训练出对应的模型文件。面向端侧进行模型设计时,需要考虑模型大小和计算量。
-
模型部署阶段:
-
模型转换:如果是Caffe, TensorFlow或ONNX平台训练的模型,需要使用X2Paddle工具将模型转换到飞桨的格式。
-
(可选步骤)模型压缩:主要优化模型大小,借助PaddleSlim提供的剪枝、量化等手段降低模型大小,以便在端上使用。
-
将模型部署到Paddle Lite。
-
在终端上通过调用Paddle Lite提供的API接口(C++、Java、Python等API接口),完成推理相关的计算。
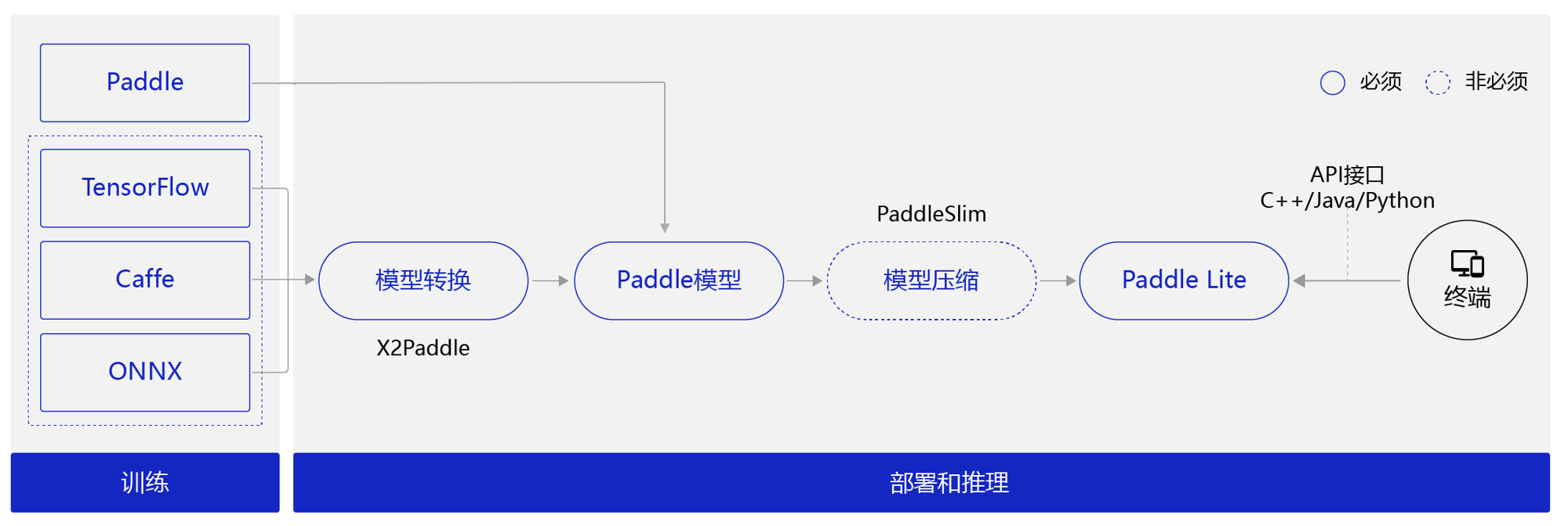
图2 推理部署流程
Paddle Lite支持的模型
Paddle Lite目前已严格验证28个模型的精度和性能,对视觉类模型做到了较为充分的支持,覆盖分类、检测、分割等多个领域,包含了特色的OCR模型的支持,并在不断丰富中。其支持的list如下:
类别
类别细分
模型
支持平台
CV
分类
MobileNetV1
ARM,X86,NPU,RKNPU,APU
CV
分类
MobileNetV2
ARM,X86,NPU
CV
分类
ResNet18
ARM,NPU
CV
分类
ResNet50
ARM,X86,NPU,XPU
CV
分类
MnasNet
ARM,NPU
CV
分类
EfficientNet*
ARM
CV
分类
SqueezeNet
ARM,NPU
CV
分类
ShufflenetV2*
ARM
CV
分类
ShuffleNet
ARM
CV
分类
InceptionV4
ARM,X86,NPU
CV
分类
VGG16
ARM
CV
分类
VGG19
XPU
CV
分类
GoogleNet
ARM,X86,XPU
CV
检测
MobileNet-SSD
ARM,NPU*
CV
检测
YOLOv3-MobileNetV3
ARM,NPU*
CV
检测
Faster R-CNN
ARM
CV
检测
Mask R-CNN*
ARM
CV
分割
Deeplabv3
ARM
CV
分割
UNet
ARM
CV
人脸
FaceDetection
ARM
CV
人脸
FaceBoxes*
ARM
CV
人脸
BlazeFace*
ARM
CV
人脸
MTCNN
ARM
CV
OCR
OCR-Attention
ARM
CV
GAN
CycleGAN*
NPU
NLP
机器翻译
Transformer*
ARM,NPU*
NLP
机器翻译
BERT
XPU
NLP
语义表示
ERNIE
XPU
注意:
-
模型列表中 * 代表该模型链接来自PaddlePaddle/models,否则为推理模型的下载链接
-
支持平台列表中 NPU* 代表ARM+NPU异构计算,否则为NPU计算
Paddle Lite部署模型工作流
使用Paddle Lite部署模型包括如下步骤:
-
准备Paddle Lite推理库。Paddle Lite新版本发布时已提供预编译库(按照支持的硬件进行组织),因此无需进行手动编译,直接下载编译好的推理库文件即可。
-
生成和优化模型。先经过模型训练得到Paddle模型,该模型不能直接用于Paddle Lite部署,需先通过Paddle Lite的opt离线优化工具优化,然后得到Paddle Lite模型(.nb格式)。如果是Caffe、TensorFlow或ONNX平台训练的模型,需要先使用X2Paddle工具将模型转换到Paddle模型格式,再使用opt优化。在这一步骤中,主要会进行模型的轻量化处理,以取得更小的体积和更快的推理速度。
-
构建推理程序。使用前续步骤中编译出来的推理库、优化后模型文件,首先经过模型初始化,配置模型位置、线程数等参数,然后进行图像预处理,如图形转换、归一化等处理,处理好以后就可以将数据输入到模型中执行推理计算,并获得推理结果。
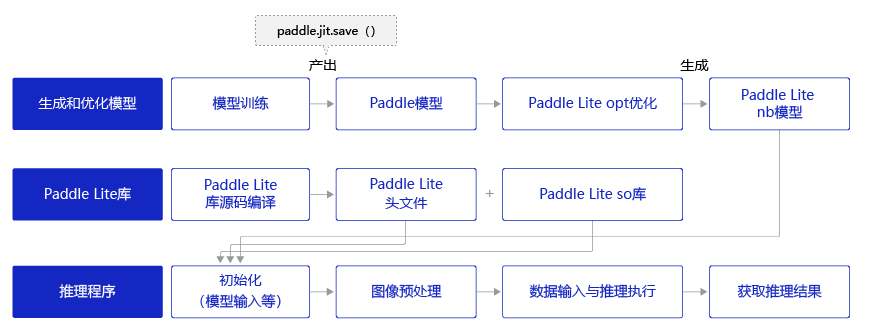
Paddle Lite移动端和嵌入端的模型部署
Paddle Lite提供多平台下的示例工程Paddle-Lite-Demo,其中包含Android、iOS和Armlinux平台,涵盖人脸识别、人像分割、图像分类、目标检测、基于视频流的人脸检测+口罩识别多个应用场景。
以Android平台为例,Paddle Lite部署的流程是:
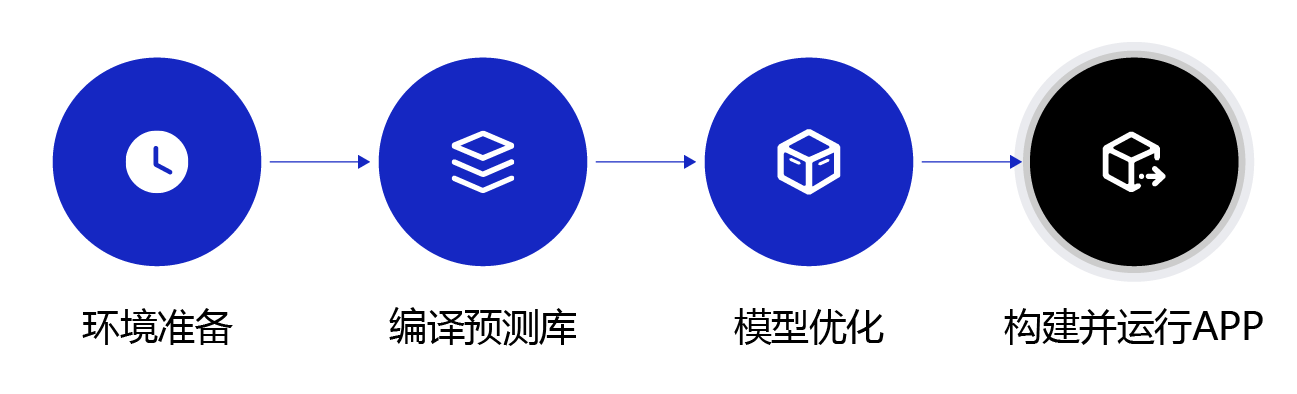
-
准备推理库。一般有两种方法:
1). 从Paddle Lite预编译库网页下载推理库文件,供示例程序调用Paddle Lite完成推理。
2). 下载Padddle Lite源码后,进行根据硬件部署环境需求,编译推理库。Paddle Lite文档上提供了不同平台的编译方法。详情请查阅:
源码编译环境准备、安卓的编译、iOS编译、ARMLinux编译。飞桨已经提供了各个主流系统和硬件的预编译库,优先推荐读者下载适合型号的预编译库,而不是自行编译,节省时间和精力。
-
模型优化。使用离线优化工具对模型进行优化,如算子融合、内存复用、类型推断、模型格式变换等,模型格式从paddle的模型格式变成paddle lite的模型格式。
-
构建并运行APP。使用前续步骤中编译出来的推理库、优化模型,完成Android/iOS平台上的目标检测应用。我们已为用户准备好了完整的Android/iOS工程示例,方便用户体验和二次开发。
Android Demo的代码结构如下图所示:
在上述Project代码结构中,有几个代码文件是比较关键的。如果想在Demo的基础上,换新的模型或者改变应用模型的方式,只要修改Predicotr.java和model.nb就可以看到实验效果。
飞桨模型压缩工具PaddleSlim
从Paddle Lite介绍中提到可以结合PaddleSlim对模型进行压缩和量化,以达到更好的性能,PaddleSlim是飞桨开源的模型压缩工具库,包含模型剪裁、定点量化、知识蒸馏、超参搜索和模型结构搜索等一系列模型压缩策略,专注于模型小型化技术。
为什么需要模型压缩
理论上来说,深度神经网络模型越深,非线性程度也就越大,相应的对现实问题的表达能力越强,但是相对应的代价是,训练成本和模型大小的增加,大模型在部署时需要更好的硬件支持,并且预测速度较低。 而随着AI应用越来越多的在手机端、IoT端上部署,这种部署环境给我们的AI模型提出了新的挑战,受能耗和设备体积的限制,端侧硬件的计算性能和存储能力相对较弱,突出的诉求主要体现在以下三点:
-
首先是速度,比如人脸闸机、人脸解锁手机等,对响应速度比较敏感,需要做到实时响应。
-
其次是存储,比如电网周边环境监测这个场景,图像目标检测模型部署在监控设备上,可用的内存只有200M。在运行了监控程序后,剩余的内存已经不到30M。
-
最后是能耗,离线翻译这种移动设备内置AI模型的能耗直接决定了它的续航能力。 以上诉求都需要我们根据终端环境对现有模型进行小型化处理,在不损失精度的情况下,让模型的体积更小、速度更快,能耗更低。
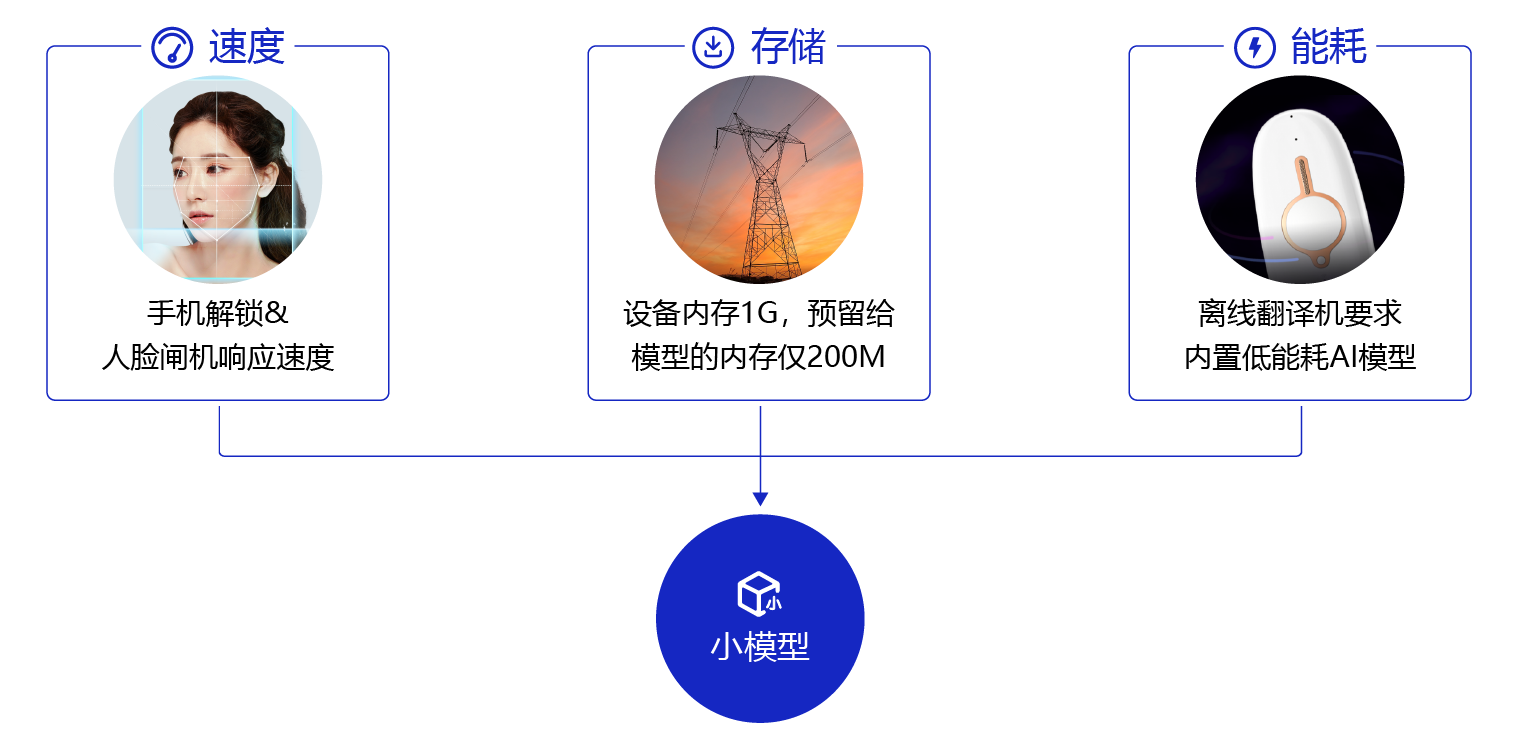
如何产出小模型? 常见的方式包括设计更高效的网络结构、将模型的参数量变少、将模型的计算量减少,同时提高模型的精度。 可能有人会提出疑问,为什么不直接设计一个小模型? 因为实际业务场景众多,人工设计有效小模型难度很高,需要设计者有非常强的领域知识。同时,小模型与大模型相比,更难以得到良好的训练。模型压缩可以在经典小模型的基础上,稍作处理就可以快速拔高模型的各项性能,达到“多快好省”的目的。
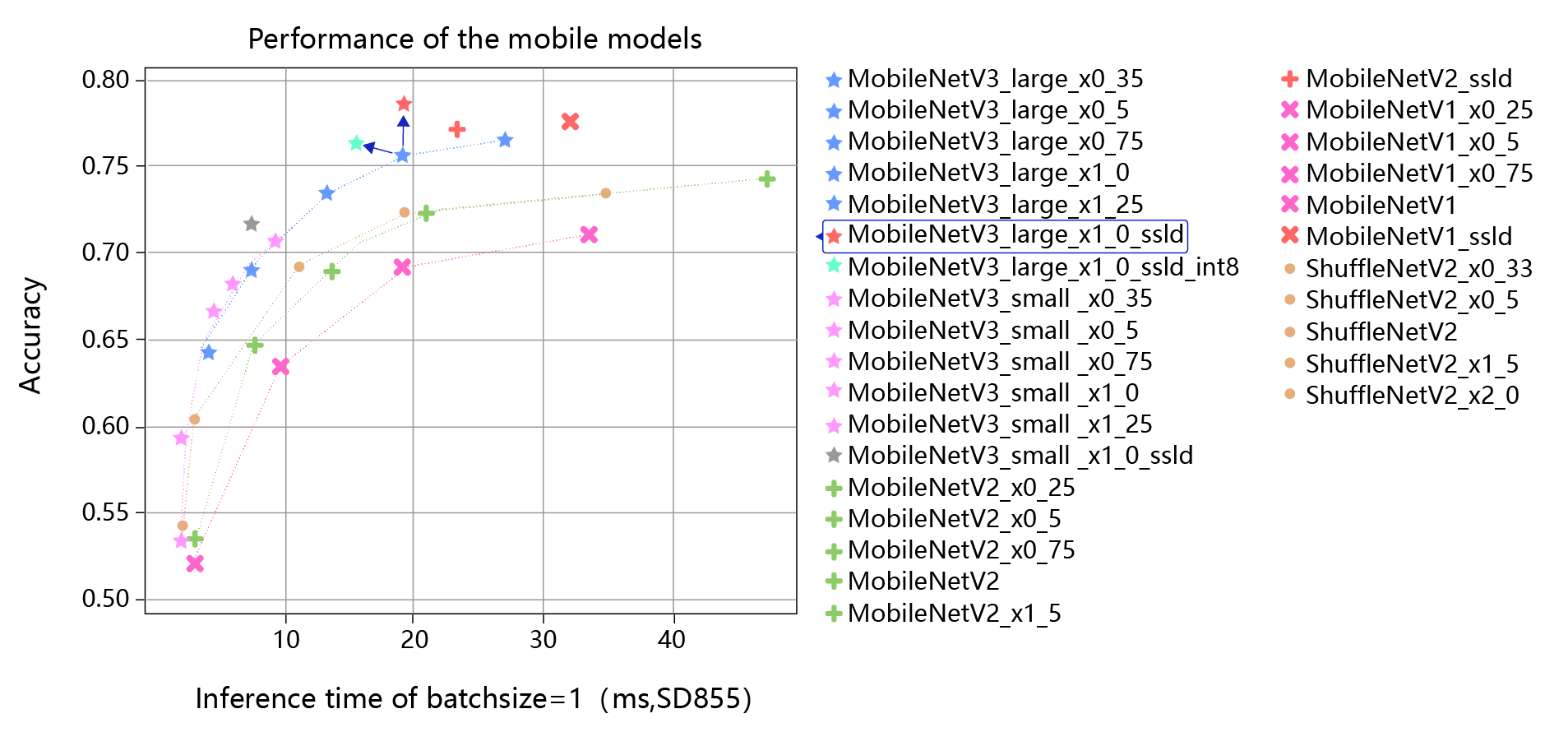
上图是分类模型使用了蒸馏和量化的效果图,横轴是推理耗时,纵轴是模型准确率。 图中最上边红色的星星对应的是在MobileNetV3_large model基础上,使用蒸馏后的效果,相比它正下方的蓝色星星,精度有明显的提升。 图中所标浅蓝色的星星,对应的是在MobileNetV3_large model基础上,使用了蒸馏和量化的结果,相比原始模型,精度和推理速度都有明显的提升。 可以看出,在人工设计的经典小模型基础上,经过蒸馏和量化可以进一步提升模型的精度和推理速度。
PaddleSlim如何实现模型压缩
PaddleSlim可以对训练好的模型进行压缩,压缩后的模型更小,并且精度几乎无损。在移动端和嵌入端,更小的模型意味着对内存的需求更小,预测速度更快。
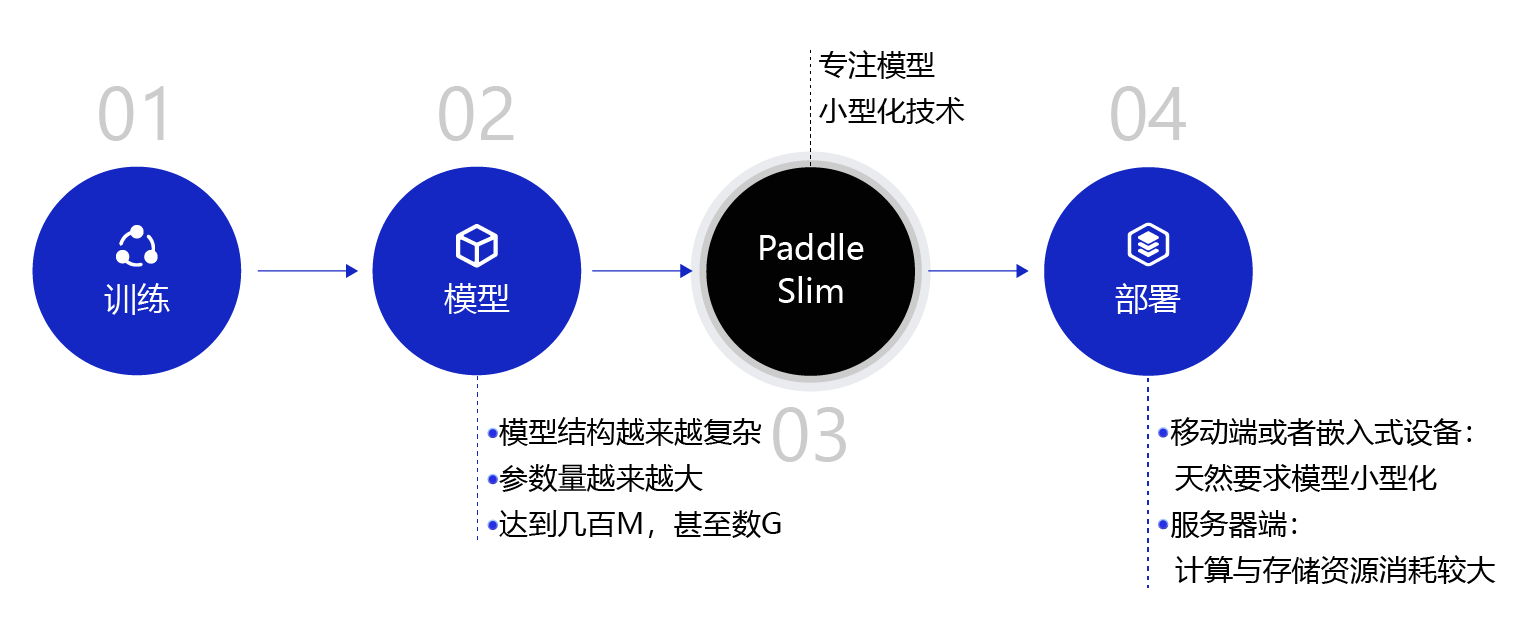
PaddleSlim提供了一站式的模型压缩算法:
-
对于业务用户,PaddleSlim提供完整的模型压缩解决方案,可用于图像分类、检测、分割等各种类型的视觉场景。 同时也在持续探索NLP领域模型的压缩方案。另外,PaddleSlim提供且在不断完善各种压缩策略在经典开源任务的benchmark, 以便业务用户参考。
-
对于模型压缩算法研究者或开发者,PaddleSlim提供各种压缩策略的底层辅助接口,方便用户复现、调研和使用最新论文方法。 PaddleSlim会从底层能力、技术咨询合作和业务场景等角度支持开发者进行模型压缩策略相关的创新工作。
PaddleSlim提供了如下模型压缩功能:
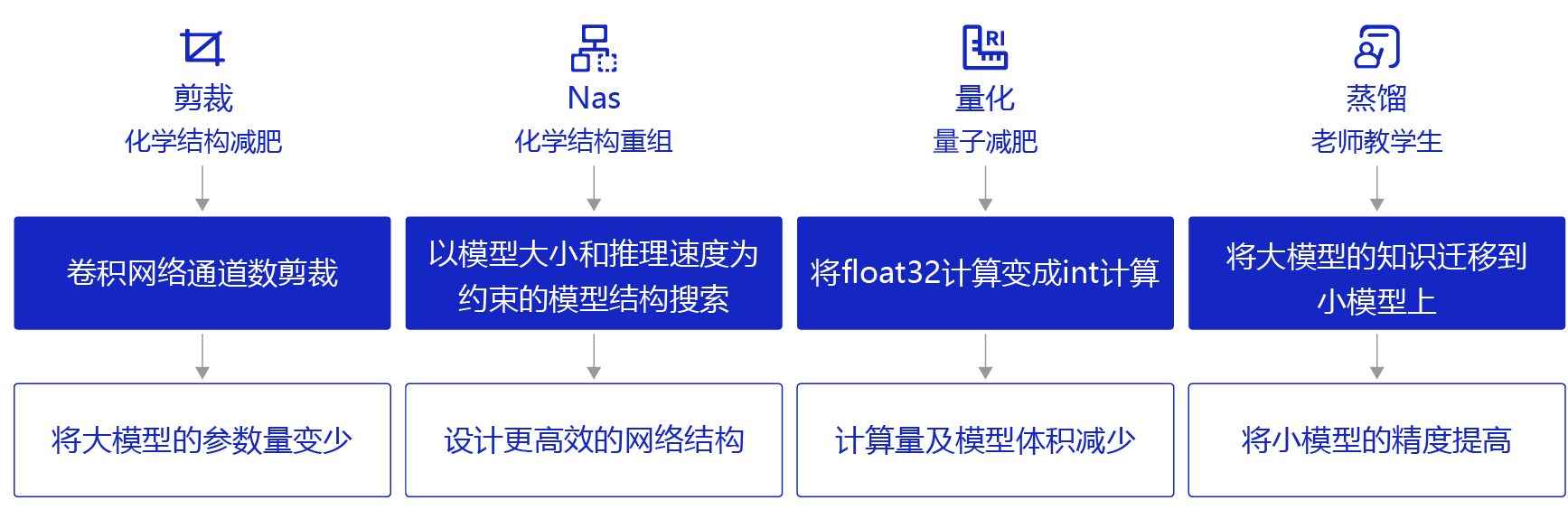
-
剪裁:类似“化学结构式的减肥”,裁剪掉一些对预测结果不重要的网络结构,网络结构变得更加“瘦身”。PaddleSlim支持按照卷积通道均匀剪裁,也支持基于敏感度的卷积通道剪裁,或基于进化算法的自动剪裁。
-
神经网络结构自动搜索(NAS):类似“化学结构式的重构”,在现有网络之上搜索出一个更精简更优秀的网络结构,支持基于进化算法的轻量神经网络结构、One-Shot网络结构等多种自动搜索策略,甚至用户可以自定义搜索算法。
-
量化:类似“量子级别的减肥”,例如将float32的数据计算精度变成int8的计算精度,在更快计算的同时,不过多降低模型效果,每个计算操作的原子变得“瘦身”。PaddleSlim既支持在线量化训练(Quantization Aware Training),也支持离线量化训练(Post-training quantization)。
-
蒸馏:类似“老师教学生”,使用一个效果好的大模型指导一个小模型训练,因为大模型可以提供更多的软分类信息量,所以会训练出一个效果接近大模型的小模型。PaddleSlim既支持单进程知识蒸馏,也支持多进程分布式知识蒸馏。
飞桨具有完善的从训练到部署的一系列框架或工具,当读者完成模型的编写和训练后,如果希望将训练好的模型放到手机端或嵌入式端(如摄像头)等去运行,可以使用飞桨轻量化推理引擎Paddle Lite。
Paddle Lite支持包括手机移动端和嵌入式端在内的端侧场景,支持广泛的硬件和平台,是一个高性能、轻量级的深度学习推理引擎。除了和飞桨核心框架无缝对接外,也兼容支持其他训练框架如TensorFlow、Caffe保存的模型(通过X2Paddle工具即可将其他格式的模型转换成飞桨模型)。
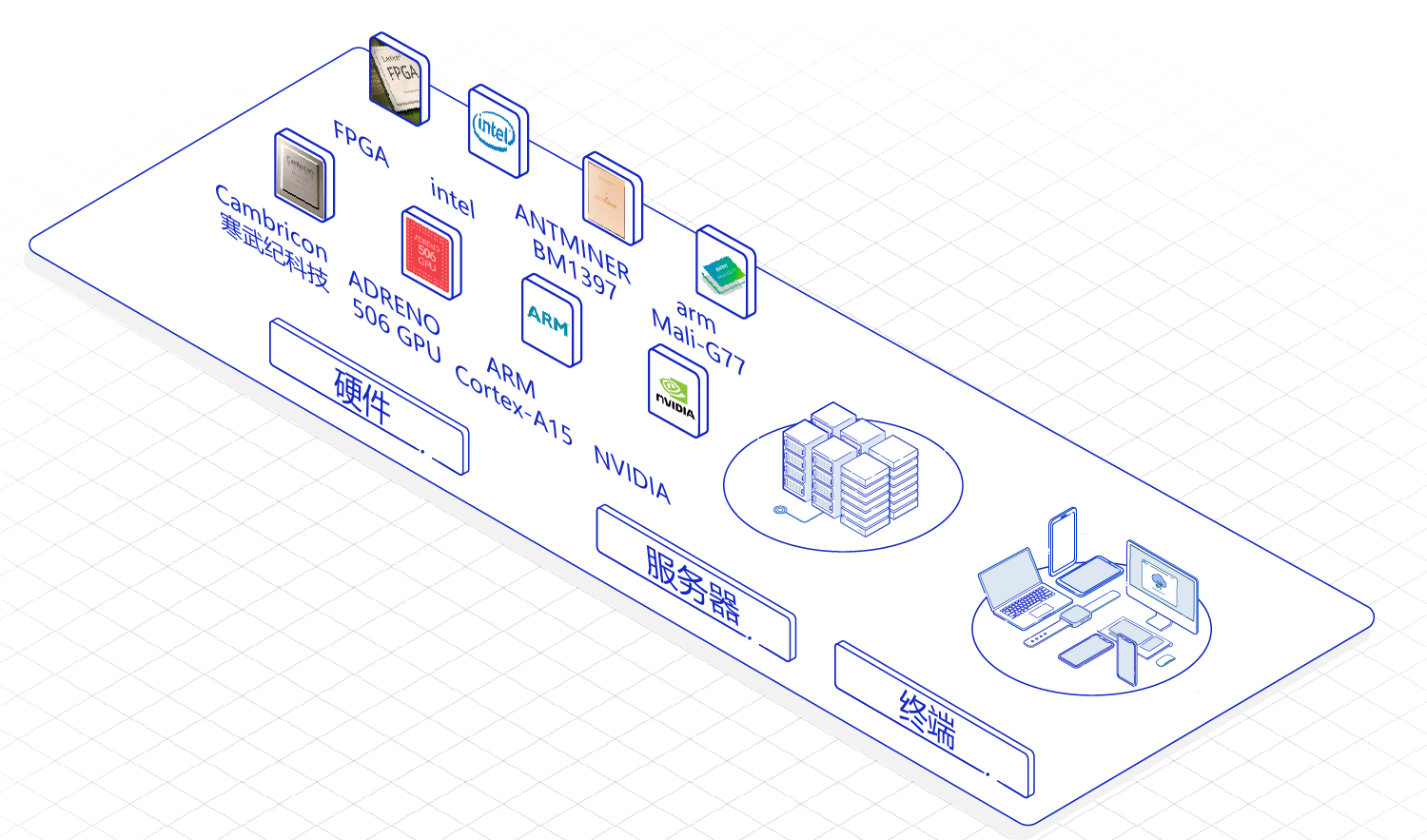
图1 多种推理终端和多种推理硬件层出不穷
随着深度学习的快速发展、特别是小型网络模型的不断成熟,原本应用到云端的深度学习推理,就可以放到终端上来做,比如手机、手表、摄像头、传感器、音响,也就是端智能。此外,可用于深度学习计算的硬件也有井喷之势,从Intel到NVIDIA、ARM、寒武纪等等。相比服务端智能,端智能具有延时低、节省资源、保护数据隐私等优势。目前已经在AI摄像、视觉特效等场景广泛应用。
然而,深度学习推理场景中,多样的平台、不同的芯片对推理库的能力提出了更高的要求。端侧模型的推理经常面临算力和内存的限制,加上日趋异构化的硬件平台和复杂的端侧使用状况,导致端侧推理引擎的架构能力颇受挑战。端侧推理引擎是端智能应用的核心模块,需要在有限算力、有限内存等限制下,高效地利用资源,快速完成推理。因此,飞桨期望提供面向不同业务算法场景、不同训练框架、不同部署环境, 简单、高效、安全的端侧推理引擎。
Paddle Lite的产品特色
为了能够完整地支持众多的硬件架构,实现在这些硬件之上的各种人工智能应用的性能优化,飞桨提供端侧推理引擎Paddle Lite。截止到现在,Paddle Lite已广泛应用于搜索广告、手机百度、百度地图、全民小视频、小度在家等多个重要业务。
Paddle Lite具备如下产品特色:
- 移动端和嵌入端的模型部署工具,可使用其部署飞桨、TensorFlow、Caffe、ONNX等多种平台的主流模型格式,包括MobileNetV1、YOLOv3、UNet、SqueezeNet等主流模型;
- 多种语言的API接口:C++/Java/Python,便于嵌入各种业务程序;
- 丰富的端侧模型:ResNet、EffcientNet、ShuffleNet、MobileNet、Unet、Face Detection、OCR_Attention等;注意因为Lite为了缩小推理库的体积,支持的算子是相对有限的,不像Paddle Inference一样支持Paddle框架的所有算子。但在移动端应用的主流轻量级模型均是支持的,可以放心使用。
- 支持丰富的移动和嵌入端芯片:ARM CPU、Mali GPU、Adreno GPU,昇腾&麒麟NPU,MTK NeuroPilot,RK NPU,MediaTek APU、寒武纪NPU,X86 CPU,NVIDIA GPU,FPGA等多种硬件平台;
- 除了Paddle Lite本身提供的性能优化策略外,还可以结合PaddleSlim可以对模型进行压缩和量化,以达到更好的性能。
Paddle Lite推理部署流程
使用Paddle Lite对模型进行推理部署的流程分两个阶段:
- 模型训练阶段:主要解决模型训练,利用标注数据训练出对应的模型文件。面向端侧进行模型设计时,需要考虑模型大小和计算量。
- 模型部署阶段:
- 模型转换:如果是Caffe, TensorFlow或ONNX平台训练的模型,需要使用X2Paddle工具将模型转换到飞桨的格式。
- (可选步骤)模型压缩:主要优化模型大小,借助PaddleSlim提供的剪枝、量化等手段降低模型大小,以便在端上使用。
- 将模型部署到Paddle Lite。
- 在终端上通过调用Paddle Lite提供的API接口(C++、Java、Python等API接口),完成推理相关的计算。
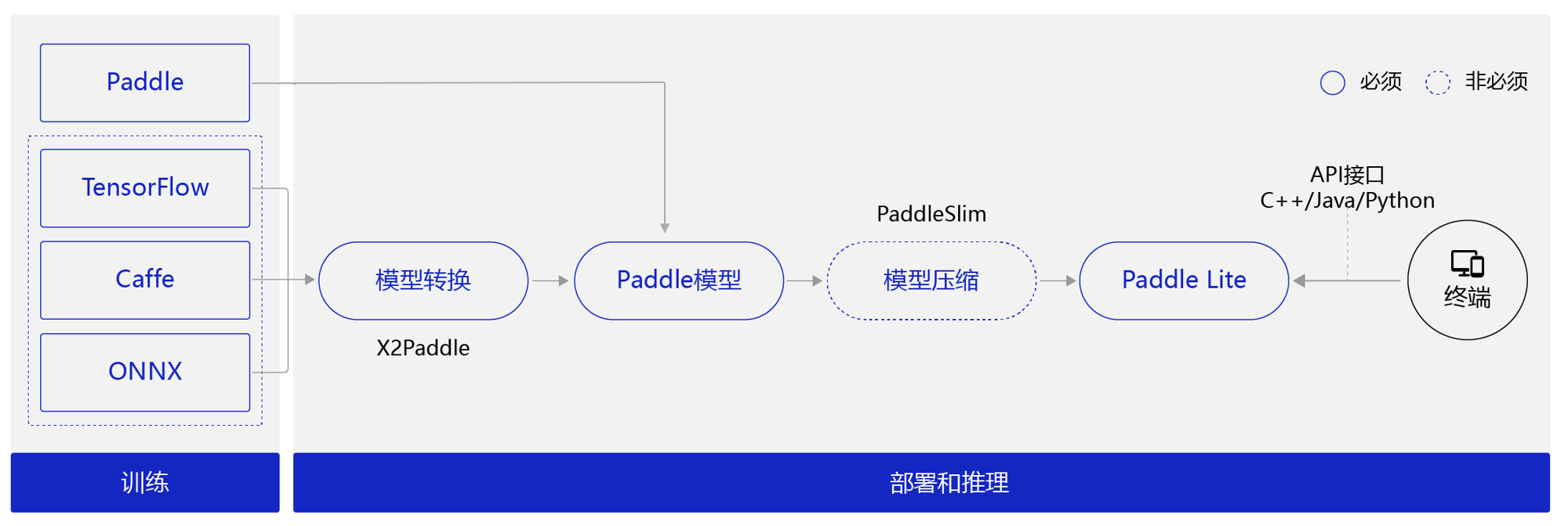
图2 推理部署流程
Paddle Lite支持的模型
Paddle Lite目前已严格验证28个模型的精度和性能,对视觉类模型做到了较为充分的支持,覆盖分类、检测、分割等多个领域,包含了特色的OCR模型的支持,并在不断丰富中。其支持的list如下:
类别 | 类别细分 | 模型 | 支持平台 |
---|---|---|---|
CV | 分类 | MobileNetV1 | ARM,X86,NPU,RKNPU,APU |
CV | 分类 | MobileNetV2 | ARM,X86,NPU |
CV | 分类 | ResNet18 | ARM,NPU |
CV | 分类 | ResNet50 | ARM,X86,NPU,XPU |
CV | 分类 | MnasNet | ARM,NPU |
CV | 分类 | EfficientNet* | ARM |
CV | 分类 | SqueezeNet | ARM,NPU |
CV | 分类 | ShufflenetV2* | ARM |
CV | 分类 | ShuffleNet | ARM |
CV | 分类 | InceptionV4 | ARM,X86,NPU |
CV | 分类 | VGG16 | ARM |
CV | 分类 | VGG19 | XPU |
CV | 分类 | GoogleNet | ARM,X86,XPU |
CV | 检测 | MobileNet-SSD | ARM,NPU* |
CV | 检测 | YOLOv3-MobileNetV3 | ARM,NPU* |
CV | 检测 | Faster R-CNN | ARM |
CV | 检测 | Mask R-CNN* | ARM |
CV | 分割 | Deeplabv3 | ARM |
CV | 分割 | UNet | ARM |
CV | 人脸 | FaceDetection | ARM |
CV | 人脸 | FaceBoxes* | ARM |
CV | 人脸 | BlazeFace* | ARM |
CV | 人脸 | MTCNN | ARM |
CV | OCR | OCR-Attention | ARM |
CV | GAN | CycleGAN* | NPU |
NLP | 机器翻译 | Transformer* | ARM,NPU* |
NLP | 机器翻译 | BERT | XPU |
NLP | 语义表示 | ERNIE | XPU |
注意:
- 模型列表中 * 代表该模型链接来自PaddlePaddle/models,否则为推理模型的下载链接
- 支持平台列表中 NPU* 代表ARM+NPU异构计算,否则为NPU计算
Paddle Lite部署模型工作流
使用Paddle Lite部署模型包括如下步骤:
- 准备Paddle Lite推理库。Paddle Lite新版本发布时已提供预编译库(按照支持的硬件进行组织),因此无需进行手动编译,直接下载编译好的推理库文件即可。
- 生成和优化模型。先经过模型训练得到Paddle模型,该模型不能直接用于Paddle Lite部署,需先通过Paddle Lite的opt离线优化工具优化,然后得到Paddle Lite模型(.nb格式)。如果是Caffe、TensorFlow或ONNX平台训练的模型,需要先使用X2Paddle工具将模型转换到Paddle模型格式,再使用opt优化。在这一步骤中,主要会进行模型的轻量化处理,以取得更小的体积和更快的推理速度。
- 构建推理程序。使用前续步骤中编译出来的推理库、优化后模型文件,首先经过模型初始化,配置模型位置、线程数等参数,然后进行图像预处理,如图形转换、归一化等处理,处理好以后就可以将数据输入到模型中执行推理计算,并获得推理结果。
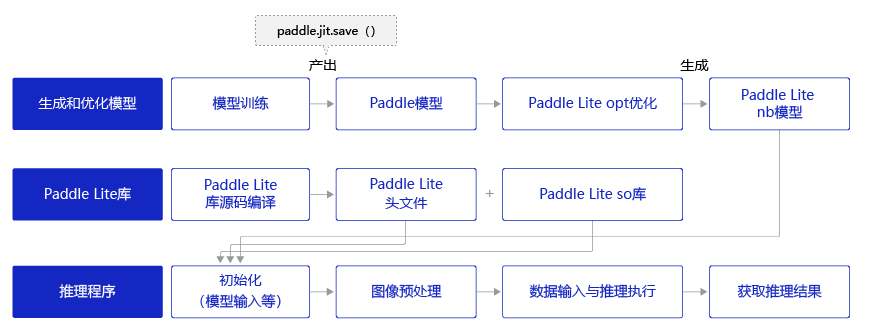
Paddle Lite移动端和嵌入端的模型部署
Paddle Lite提供多平台下的示例工程Paddle-Lite-Demo,其中包含Android、iOS和Armlinux平台,涵盖人脸识别、人像分割、图像分类、目标检测、基于视频流的人脸检测+口罩识别多个应用场景。
以Android平台为例,Paddle Lite部署的流程是:
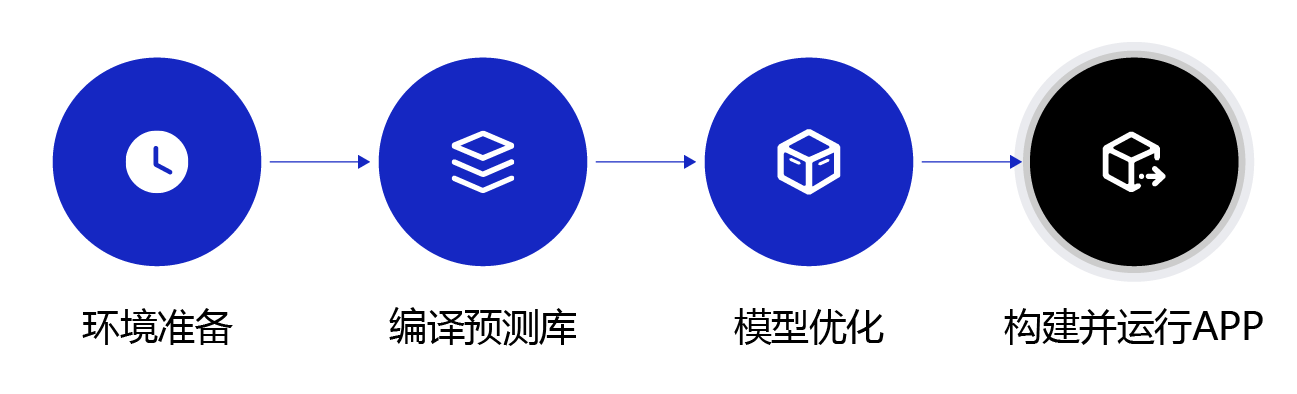
-
准备推理库。一般有两种方法:
1). 从Paddle Lite预编译库网页下载推理库文件,供示例程序调用Paddle Lite完成推理。
2). 下载Padddle Lite源码后,进行根据硬件部署环境需求,编译推理库。Paddle Lite文档上提供了不同平台的编译方法。详情请查阅:
源码编译环境准备、安卓的编译、iOS编译、ARMLinux编译。飞桨已经提供了各个主流系统和硬件的预编译库,优先推荐读者下载适合型号的预编译库,而不是自行编译,节省时间和精力。 - 模型优化。使用离线优化工具对模型进行优化,如算子融合、内存复用、类型推断、模型格式变换等,模型格式从paddle的模型格式变成paddle lite的模型格式。
- 构建并运行APP。使用前续步骤中编译出来的推理库、优化模型,完成Android/iOS平台上的目标检测应用。我们已为用户准备好了完整的Android/iOS工程示例,方便用户体验和二次开发。
Android Demo的代码结构如下图所示:
在上述Project代码结构中,有几个代码文件是比较关键的。如果想在Demo的基础上,换新的模型或者改变应用模型的方式,只要修改Predicotr.java和model.nb就可以看到实验效果。
飞桨模型压缩工具PaddleSlim
从Paddle Lite介绍中提到可以结合PaddleSlim对模型进行压缩和量化,以达到更好的性能,PaddleSlim是飞桨开源的模型压缩工具库,包含模型剪裁、定点量化、知识蒸馏、超参搜索和模型结构搜索等一系列模型压缩策略,专注于模型小型化技术。
为什么需要模型压缩
理论上来说,深度神经网络模型越深,非线性程度也就越大,相应的对现实问题的表达能力越强,但是相对应的代价是,训练成本和模型大小的增加,大模型在部署时需要更好的硬件支持,并且预测速度较低。 而随着AI应用越来越多的在手机端、IoT端上部署,这种部署环境给我们的AI模型提出了新的挑战,受能耗和设备体积的限制,端侧硬件的计算性能和存储能力相对较弱,突出的诉求主要体现在以下三点:
- 首先是速度,比如人脸闸机、人脸解锁手机等,对响应速度比较敏感,需要做到实时响应。
- 其次是存储,比如电网周边环境监测这个场景,图像目标检测模型部署在监控设备上,可用的内存只有200M。在运行了监控程序后,剩余的内存已经不到30M。
- 最后是能耗,离线翻译这种移动设备内置AI模型的能耗直接决定了它的续航能力。 以上诉求都需要我们根据终端环境对现有模型进行小型化处理,在不损失精度的情况下,让模型的体积更小、速度更快,能耗更低。
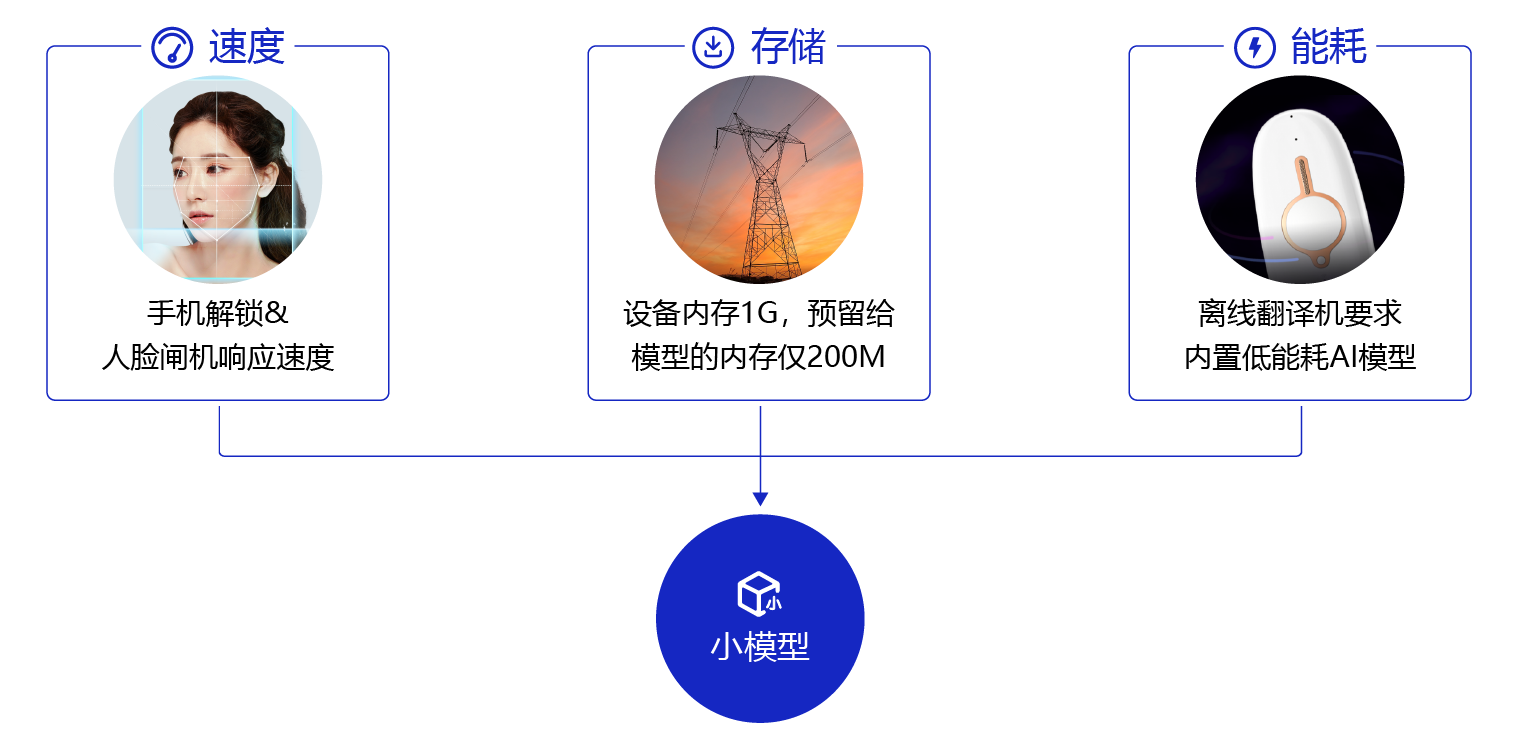
如何产出小模型? 常见的方式包括设计更高效的网络结构、将模型的参数量变少、将模型的计算量减少,同时提高模型的精度。 可能有人会提出疑问,为什么不直接设计一个小模型? 因为实际业务场景众多,人工设计有效小模型难度很高,需要设计者有非常强的领域知识。同时,小模型与大模型相比,更难以得到良好的训练。模型压缩可以在经典小模型的基础上,稍作处理就可以快速拔高模型的各项性能,达到“多快好省”的目的。
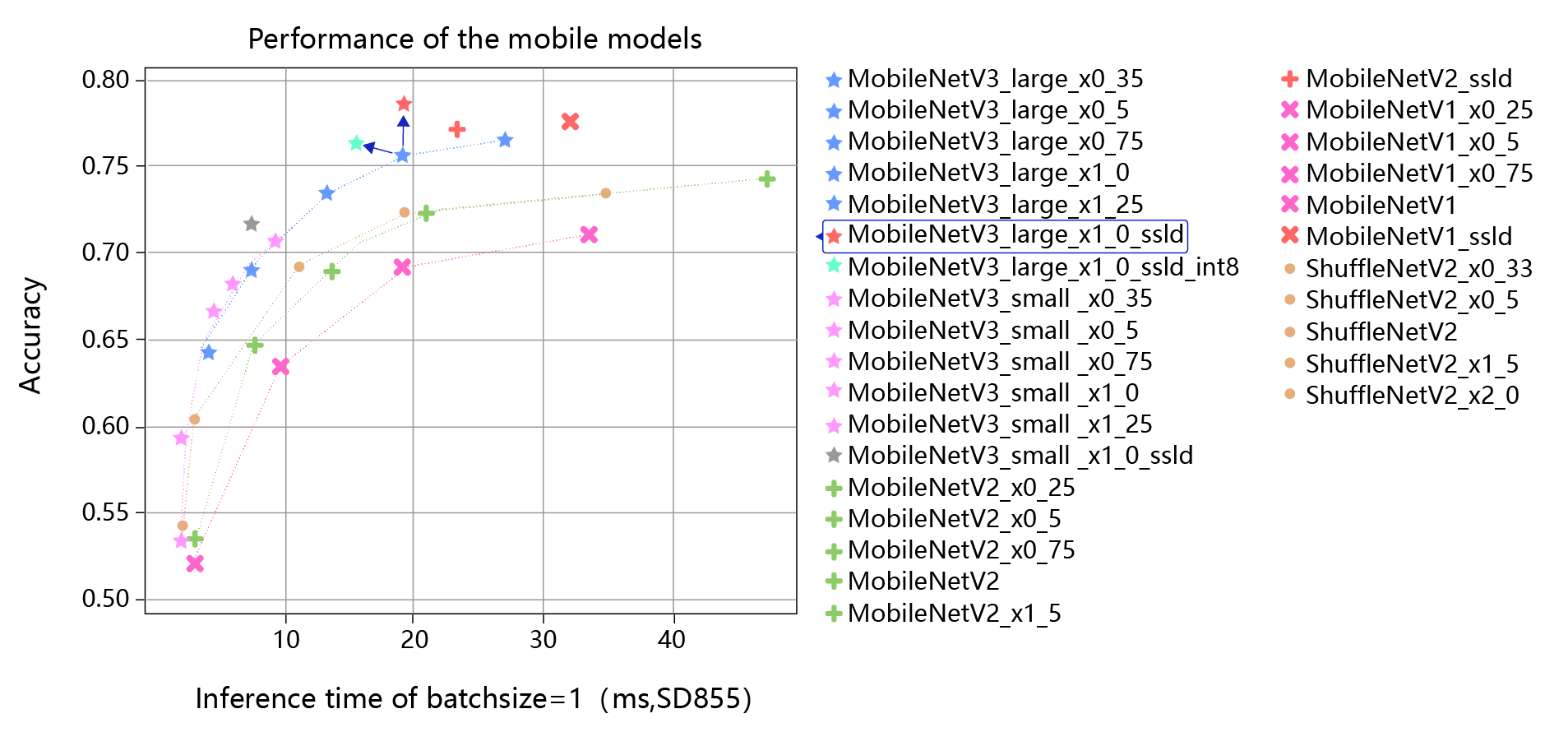
上图是分类模型使用了蒸馏和量化的效果图,横轴是推理耗时,纵轴是模型准确率。 图中最上边红色的星星对应的是在MobileNetV3_large model基础上,使用蒸馏后的效果,相比它正下方的蓝色星星,精度有明显的提升。 图中所标浅蓝色的星星,对应的是在MobileNetV3_large model基础上,使用了蒸馏和量化的结果,相比原始模型,精度和推理速度都有明显的提升。 可以看出,在人工设计的经典小模型基础上,经过蒸馏和量化可以进一步提升模型的精度和推理速度。
PaddleSlim如何实现模型压缩
PaddleSlim可以对训练好的模型进行压缩,压缩后的模型更小,并且精度几乎无损。在移动端和嵌入端,更小的模型意味着对内存的需求更小,预测速度更快。
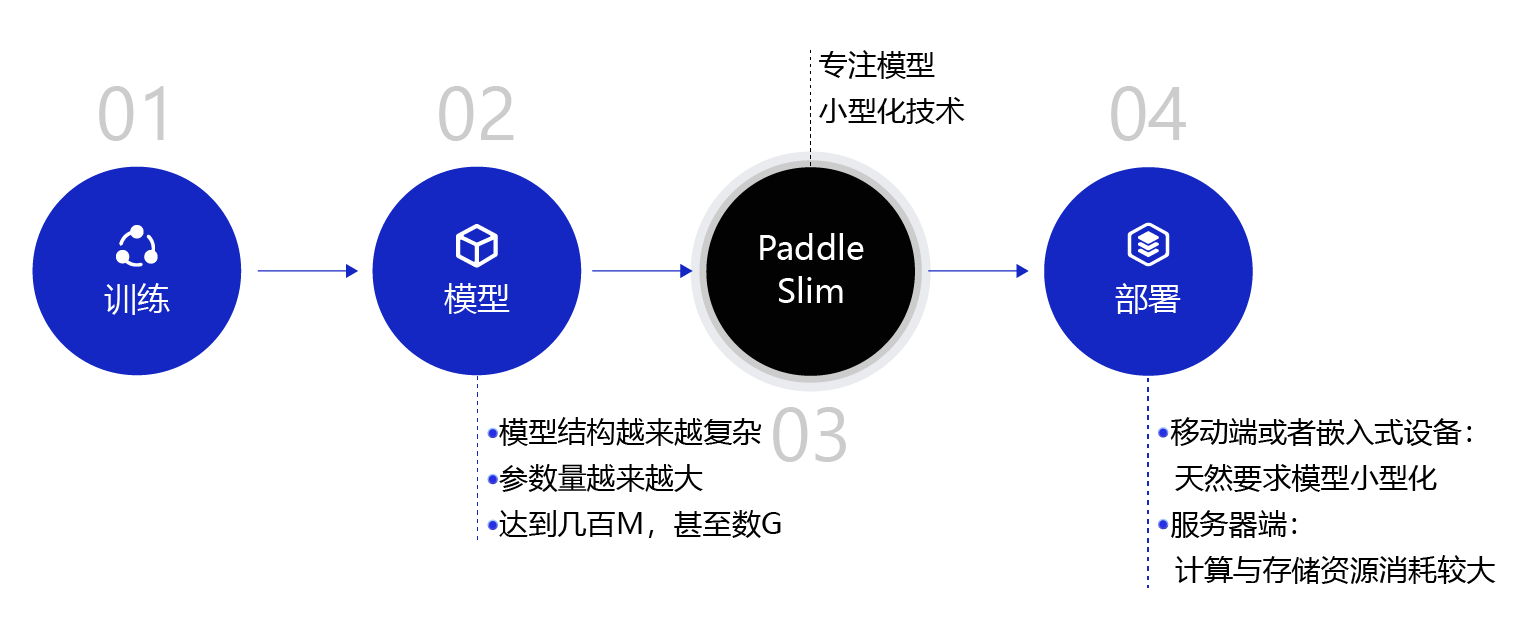
PaddleSlim提供了一站式的模型压缩算法:
- 对于业务用户,PaddleSlim提供完整的模型压缩解决方案,可用于图像分类、检测、分割等各种类型的视觉场景。 同时也在持续探索NLP领域模型的压缩方案。另外,PaddleSlim提供且在不断完善各种压缩策略在经典开源任务的benchmark, 以便业务用户参考。
- 对于模型压缩算法研究者或开发者,PaddleSlim提供各种压缩策略的底层辅助接口,方便用户复现、调研和使用最新论文方法。 PaddleSlim会从底层能力、技术咨询合作和业务场景等角度支持开发者进行模型压缩策略相关的创新工作。
PaddleSlim提供了如下模型压缩功能:
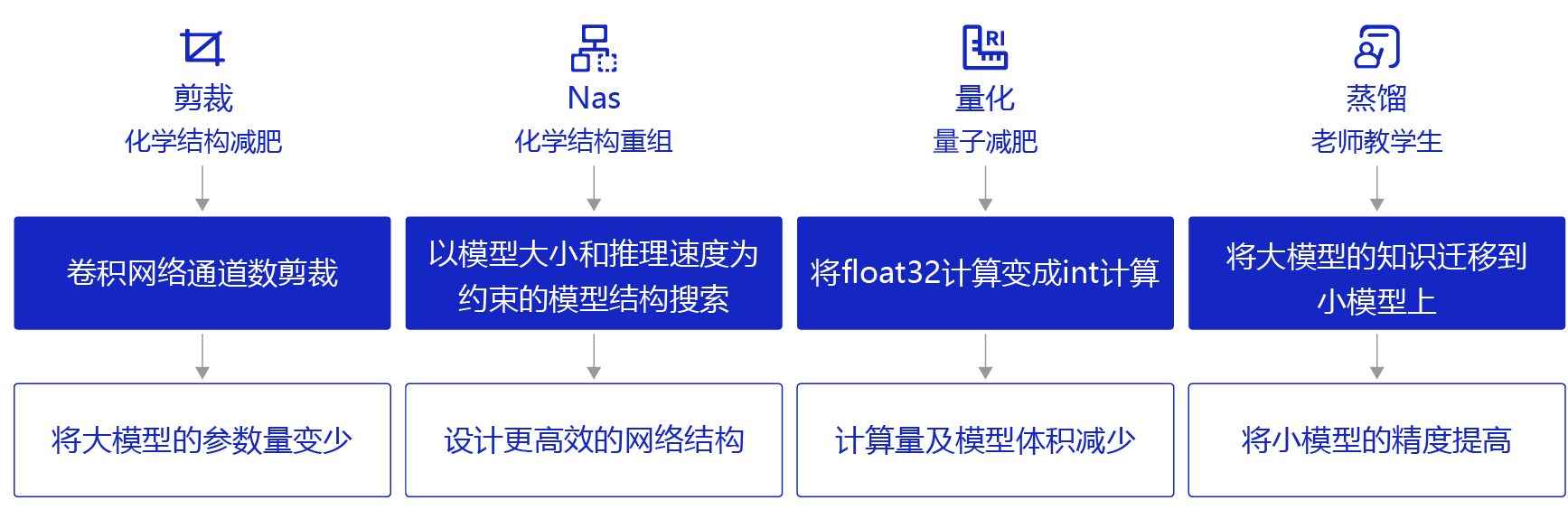
- 剪裁:类似“化学结构式的减肥”,裁剪掉一些对预测结果不重要的网络结构,网络结构变得更加“瘦身”。PaddleSlim支持按照卷积通道均匀剪裁,也支持基于敏感度的卷积通道剪裁,或基于进化算法的自动剪裁。
- 神经网络结构自动搜索(NAS):类似“化学结构式的重构”,在现有网络之上搜索出一个更精简更优秀的网络结构,支持基于进化算法的轻量神经网络结构、One-Shot网络结构等多种自动搜索策略,甚至用户可以自定义搜索算法。
- 量化:类似“量子级别的减肥”,例如将float32的数据计算精度变成int8的计算精度,在更快计算的同时,不过多降低模型效果,每个计算操作的原子变得“瘦身”。PaddleSlim既支持在线量化训练(Quantization Aware Training),也支持离线量化训练(Post-training quantization)。
- 蒸馏:类似“老师教学生”,使用一个效果好的大模型指导一个小模型训练,因为大模型可以提供更多的软分类信息量,所以会训练出一个效果接近大模型的小模型。PaddleSlim既支持单进程知识蒸馏,也支持多进程分布式知识蒸馏。
PaddleSlim模型剪裁
下面以MobileNetV1模型和Cifar10分类任务为例,介绍如何使用PaddleSlim对动态图卷积模型进行剪裁,其他模型压缩方法的使用可以参考PaddleSlim的文档。
1. 模型定义
PaddlePaddle提供的vision模块提供了一些构建好的分类模型结构,并提供在ImageNet数据集上的预训练模型。为了简化教程,我们不再重新定义网络结构,而是直接从vision模块导入模型结构。代码如下所示,我们导入MobileNetV1模型,并查看模型的结构信息。
注意:使用以下方法安装PaddleSlim后,需要重启代码执行器,以便重新加载python模块。
# 安装或更新PaddlePaddlePaddle到2.0.0版本 # 注意:如果是GPU环境,需要安装GPU版本的PaddlePaddle
#!python -m pip install paddlepaddle==2.0.0 #!python -m pip install paddlepaddle-gpu==2.0.0
#您可以通过pip来安装 #如果编译安装PaddleSlim2.0.0, 安装完后需要重启代码执行器 #rm -rf PaddleSlim
#!git clone https://github.com/PaddlePaddle/PaddleSlim.git && cd PaddleSlim && git checkout remotes
/origin/release/2.0.0 && python setup.py install !python -m pip install paddleslim==2.0.0
Looking in indexes: https://pypi.tuna.tsinghua.edu.cn/simple Collecting paddleslim==2.0.0 Downloading https://pypi.tuna.tsinghua.edu.cn/packages/f5/72/567086025f68b20223412ddd444c23c
d3a825288750b9d8699fdd424b751/paddleslim-2.0.0-py2.py3-none-any.whl (297 kB) ━━━━━━━━━
━━━━━━━━━━━━━━━━━━━━━━━━━━━━━━ 297.8/297.8 KB 3.4 MB/s eta 0:00:00a
0:00:01 Requirement already satisfied: tqdm in /opt/conda/envs/python35-paddle120-env/lib/python
3.7/site-packages (from paddleslim==2.0.0) (4.27.0) Requirement already satisfied: pillow in /opt/conda/envs/python35-paddle120-env/lib/python3.7
/site-packages (from paddleslim==2.0.0) (8.2.0) Requirement already satisfied: pyzmq in /opt/conda/envs/python35-paddle120-env/lib/python3.7/s
ite-packages (from paddleslim==2.0.0) (22.3.0) Requirement already satisfied: matplotlib in /opt/conda/envs/python35-paddle120-env/lib/python3
.7/site-packages (from paddleslim==2.0.0) (2.2.3) Requirement already satisfied: opencv-python in /opt/conda/envs/python35-paddle120-env/lib/pyth
on3.7/site-packages (from paddleslim==2.0.0) (4.1.1.26) Requirement already satisfied: python-dateutil>=2.1 in /opt/conda/envs/python35-paddle120-env
/lib/python3.7/site-packages (from matplotlib->paddleslim==2.0.0) (2.8.2) Requirement already satisfied: cycler>=0.10 in /opt/conda/envs/python35-paddle120-env/lib/python
3.7/site-packages (from matplotlib->paddleslim==2.0.0) (0.10.0) Requirement already satisfied: numpy>=1.7.1 in /opt/conda/envs/python35-paddle120-env/lib/python
3.7/site-packages (from matplotlib->paddleslim==2.0.0) (1.19.5) Requirement already satisfied: six>=1.10 in /opt/conda/envs/python35-paddle120-env/lib/python3.7
/site-packages (from matplotlib->paddleslim==2.0.0) (1.16.0) Requirement already satisfied: pytz in /opt/conda/envs/python35-paddle120-env/lib/python3.7/
site-packages (from matplotlib->paddleslim==2.0.0) (2019.3) Requirement already satisfied: pyparsing!=2.0.4,!=2.1.2,!=2.1.6,>=2.0.1 in /opt/conda/envs/py
thon35-paddle120-env/lib/python3.7/site-packages (from matplotlib->paddleslim==2.0.0) (3.0.8) Requirement already satisfied: kiwisolver>=1.0.1 in /opt/conda/envs/python35-paddle120-env/lib
/python3.7/site-packages (from matplotlib->paddleslim==2.0.0) (1.1.0) Requirement already satisfied: setuptools in /opt/conda/envs/python35-paddle120-env/lib/python3.7
/site-packages (from kiwisolver>=1.0.1->matplotlib->paddleslim==2.0.0) (56.2.0) Installing collected packages: paddleslim Successfully installed paddleslim-2.0.0
import paddle from paddle.vision.models import mobilenet_v1 #使用预置的mobilenet模型,
但不使用预训练的参数 net = mobilenet_v1(pretrained=False)
paddle.summary(net, (1, 3, 32, 32))
W0506 16:02:16.985889 218 gpu_context.cc:244] Please NOTE: device: 0, GPU Compute Capability:
7.0, Driver API Version: 10.1, Runtime API Version: 10.1 W0506 16:02:16.992015 218 gpu_context.cc:272] device: 0, cuDNN Version: 7.6.
--------------------------------------------------------------------------------- Layer (type) Input Shape Output Shape Param # ================================================================================= Conv2D-1 [[1, 3, 32, 32]] [1, 32, 16, 16] 864 BatchNorm2D-1 [[1, 32, 16, 16]] [1, 32, 16, 16] 128 ReLU-1 [[1, 32, 16, 16]] [1, 32, 16, 16] 0 ConvBNLayer-1 [[1, 3, 32, 32]] [1, 32, 16, 16] 0 Conv2D-2 [[1, 32, 16, 16]] [1, 32, 16, 16] 288 BatchNorm2D-2 [[1, 32, 16, 16]] [1, 32, 16, 16] 128 ReLU-2 [[1, 32, 16, 16]] [1, 32, 16, 16] 0 ConvBNLayer-2 [[1, 32, 16, 16]] [1, 32, 16, 16] 0 Conv2D-3 [[1, 32, 16, 16]] [1, 64, 16, 16] 2,048 BatchNorm2D-3 [[1, 64, 16, 16]] [1, 64, 16, 16] 256 ReLU-3 [[1, 64, 16, 16]] [1, 64, 16, 16] 0 ConvBNLayer-3 [[1, 32, 16, 16]] [1, 64, 16, 16] 0 DepthwiseSeparable-1 [[1, 32, 16, 16]] [1, 64, 16, 16] 0 Conv2D-4 [[1, 64, 16, 16]] [1, 64, 8, 8] 576 BatchNorm2D-4 [[1, 64, 8, 8]] [1, 64, 8, 8] 256 ReLU-4 [[1, 64, 8, 8]] [1, 64, 8, 8] 0 ConvBNLayer-4 [[1, 64, 16, 16]] [1, 64, 8, 8] 0 Conv2D-5 [[1, 64, 8, 8]] [1, 128, 8, 8] 8,192 BatchNorm2D-5 [[1, 128, 8, 8]] [1, 128, 8, 8] 512 ReLU-5 [[1, 128, 8, 8]] [1, 128, 8, 8] 0 ConvBNLayer-5 [[1, 64, 8, 8]] [1, 128, 8, 8] 0 DepthwiseSeparable-2 [[1, 64, 16, 16]] [1, 128, 8, 8] 0 Conv2D-6 [[1, 128, 8, 8]] [1, 128, 8, 8] 1,152 BatchNorm2D-6 [[1, 128, 8, 8]] [1, 128, 8, 8] 512 ReLU-6 [[1, 128, 8, 8]] [1, 128, 8, 8] 0 ConvBNLayer-6 [[1, 128, 8, 8]] [1, 128, 8, 8] 0 Conv2D-7 [[1, 128, 8, 8]] [1, 128, 8, 8] 16,384 BatchNorm2D-7 [[1, 128, 8, 8]] [1, 128, 8, 8] 512 ReLU-7 [[1, 128, 8, 8]] [1, 128, 8, 8] 0 ConvBNLayer-7 [[1, 128, 8, 8]] [1, 128, 8, 8] 0 DepthwiseSeparable-3 [[1, 128, 8, 8]] [1, 128, 8, 8] 0 Conv2D-8 [[1, 128, 8, 8]] [1, 128, 4, 4] 1,152 BatchNorm2D-8 [[1, 128, 4, 4]] [1, 128, 4, 4] 512 ReLU-8 [[1, 128, 4, 4]] [1, 128, 4, 4] 0 ConvBNLayer-8 [[1, 128, 8, 8]] [1, 128, 4, 4] 0 Conv2D-9 [[1, 128, 4, 4]] [1, 256, 4, 4] 32,768 BatchNorm2D-9 [[1, 256, 4, 4]] [1, 256, 4, 4] 1,024 ReLU-9 [[1, 256, 4, 4]] [1, 256, 4, 4] 0 ConvBNLayer-9 [[1, 128, 4, 4]] [1, 256, 4, 4] 0 DepthwiseSeparable-4 [[1, 128, 8, 8]] [1, 256, 4, 4] 0 Conv2D-10 [[1, 256, 4, 4]] [1, 256, 4, 4] 2,304 BatchNorm2D-10 [[1, 256, 4, 4]] [1, 256, 4, 4] 1,024 ReLU-10 [[1, 256, 4, 4]] [1, 256, 4, 4] 0 ConvBNLayer-10 [[1, 256, 4, 4]] [1, 256, 4, 4] 0 Conv2D-11 [[1, 256, 4, 4]] [1, 256, 4, 4] 65,536 BatchNorm2D-11 [[1, 256, 4, 4]] [1, 256, 4, 4] 1,024 ReLU-11 [[1, 256, 4, 4]] [1, 256, 4, 4] 0 ConvBNLayer-11 [[1, 256, 4, 4]] [1, 256, 4, 4] 0 DepthwiseSeparable-5 [[1, 256, 4, 4]] [1, 256, 4, 4] 0 Conv2D-12 [[1, 256, 4, 4]] [1, 256, 2, 2] 2,304 BatchNorm2D-12 [[1, 256, 2, 2]] [1, 256, 2, 2] 1,024 ReLU-12 [[1, 256, 2, 2]] [1, 256, 2, 2] 0 ConvBNLayer-12 [[1, 256, 4, 4]] [1, 256, 2, 2] 0 Conv2D-13 [[1, 256, 2, 2]] [1, 512, 2, 2] 131,072 BatchNorm2D-13 [[1, 512, 2, 2]] [1, 512, 2, 2] 2,048 ReLU-13 [[1, 512, 2, 2]] [1, 512, 2, 2] 0 ConvBNLayer-13 [[1, 256, 2, 2]] [1, 512, 2, 2] 0 DepthwiseSeparable-6 [[1, 256, 4, 4]] [1, 512, 2, 2] 0 Conv2D-14 [[1, 512, 2, 2]] [1, 512, 2, 2] 4,608 BatchNorm2D-14 [[1, 512, 2, 2]] [1, 512, 2, 2] 2,048 ReLU-14 [[1, 512, 2, 2]] [1, 512, 2, 2] 0 ConvBNLayer-14 [[1, 512, 2, 2]] [1, 512, 2, 2] 0 Conv2D-15 [[1, 512, 2, 2]] [1, 512, 2, 2] 262,144 BatchNorm2D-15 [[1, 512, 2, 2]] [1, 512, 2, 2] 2,048 ReLU-15 [[1, 512, 2, 2]] [1, 512, 2, 2] 0 ConvBNLayer-15 [[1, 512, 2, 2]] [1, 512, 2, 2] 0 DepthwiseSeparable-7 [[1, 512, 2, 2]] [1, 512, 2, 2] 0 Conv2D-16 [[1, 512, 2, 2]] [1, 512, 2, 2] 4,608 BatchNorm2D-16 [[1, 512, 2, 2]] [1, 512, 2, 2] 2,048 ReLU-16 [[1, 512, 2, 2]] [1, 512, 2, 2] 0 ConvBNLayer-16 [[1, 512, 2, 2]] [1, 512, 2, 2] 0 Conv2D-17 [[1, 512, 2, 2]] [1, 512, 2, 2] 262,144 BatchNorm2D-17 [[1, 512, 2, 2]] [1, 512, 2, 2] 2,048 ReLU-17 [[1, 512, 2, 2]] [1, 512, 2, 2] 0 ConvBNLayer-17 [[1, 512, 2, 2]] [1, 512, 2, 2] 0 DepthwiseSeparable-8 [[1, 512, 2, 2]] [1, 512, 2, 2] 0 Conv2D-18 [[1, 512, 2, 2]] [1, 512, 2, 2] 4,608 BatchNorm2D-18 [[1, 512, 2, 2]] [1, 512, 2, 2] 2,048 ReLU-18 [[1, 512, 2, 2]] [1, 512, 2, 2] 0 ConvBNLayer-18 [[1, 512, 2, 2]] [1, 512, 2, 2] 0 Conv2D-19 [[1, 512, 2, 2]] [1, 512, 2, 2] 262,144 BatchNorm2D-19 [[1, 512, 2, 2]] [1, 512, 2, 2] 2,048 ReLU-19 [[1, 512, 2, 2]] [1, 512, 2, 2] 0 ConvBNLayer-19 [[1, 512, 2, 2]] [1, 512, 2, 2] 0 DepthwiseSeparable-9 [[1, 512, 2, 2]] [1, 512, 2, 2] 0 Conv2D-20 [[1, 512, 2, 2]] [1, 512, 2, 2] 4,608 BatchNorm2D-20 [[1, 512, 2, 2]] [1, 512, 2, 2] 2,048 ReLU-20 [[1, 512, 2, 2]] [1, 512, 2, 2] 0 ConvBNLayer-20 [[1, 512, 2, 2]] [1, 512, 2, 2] 0 Conv2D-21 [[1, 512, 2, 2]] [1, 512, 2, 2] 262,144 BatchNorm2D-21 [[1, 512, 2, 2]] [1, 512, 2, 2] 2,048 ReLU-21 [[1, 512, 2, 2]] [1, 512, 2, 2] 0 ConvBNLayer-21 [[1, 512, 2, 2]] [1, 512, 2, 2] 0 DepthwiseSeparable-10 [[1, 512, 2, 2]] [1, 512, 2, 2] 0 Conv2D-22 [[1, 512, 2, 2]] [1, 512, 2, 2] 4,608 BatchNorm2D-22 [[1, 512, 2, 2]] [1, 512, 2, 2] 2,048 ReLU-22 [[1, 512, 2, 2]] [1, 512, 2, 2] 0 ConvBNLayer-22 [[1, 512, 2, 2]] [1, 512, 2, 2] 0 Conv2D-23 [[1, 512, 2, 2]] [1, 512, 2, 2] 262,144 BatchNorm2D-23 [[1, 512, 2, 2]] [1, 512, 2, 2] 2,048 ReLU-23 [[1, 512, 2, 2]] [1, 512, 2, 2] 0 ConvBNLayer-23 [[1, 512, 2, 2]] [1, 512, 2, 2] 0 DepthwiseSeparable-11 [[1, 512, 2, 2]] [1, 512, 2, 2] 0 Conv2D-24 [[1, 512, 2, 2]] [1, 512, 1, 1] 4,608 BatchNorm2D-24 [[1, 512, 1, 1]] [1, 512, 1, 1] 2,048 ReLU-24 [[1, 512, 1, 1]] [1, 512, 1, 1] 0 ConvBNLayer-24 [[1, 512, 2, 2]] [1, 512, 1, 1] 0 Conv2D-25 [[1, 512, 1, 1]] [1, 1024, 1, 1] 524,288 BatchNorm2D-25 [[1, 1024, 1, 1]] [1, 1024, 1, 1] 4,096 ReLU-25 [[1, 1024, 1, 1]] [1, 1024, 1, 1] 0 ConvBNLayer-25 [[1, 512, 1, 1]] [1, 1024, 1, 1] 0 DepthwiseSeparable-12 [[1, 512, 2, 2]] [1, 1024, 1, 1] 0 Conv2D-26 [[1, 1024, 1, 1]] [1, 1024, 1, 1] 9,216 BatchNorm2D-26 [[1, 1024, 1, 1]] [1, 1024, 1, 1] 4,096 ReLU-26 [[1, 1024, 1, 1]] [1, 1024, 1, 1] 0 ConvBNLayer-26 [[1, 1024, 1, 1]] [1, 1024, 1, 1] 0 Conv2D-27 [[1, 1024, 1, 1]] [1, 1024, 1, 1] 1,048,576 BatchNorm2D-27 [[1, 1024, 1, 1]] [1, 1024, 1, 1] 4,096 ReLU-27 [[1, 1024, 1, 1]] [1, 1024, 1, 1] 0 ConvBNLayer-27 [[1, 1024, 1, 1]] [1, 1024, 1, 1] 0 DepthwiseSeparable-13 [[1, 1024, 1, 1]] [1, 1024, 1, 1] 0 AdaptiveAvgPool2D-1 [[1, 1024, 1, 1]] [1, 1024, 1, 1] 0 Linear-1 [[1, 1024]] [1, 1000] 1,025,000 ================================================================================= Total params: 4,253,864 Trainable params: 4,210,088 Non-trainable params: 43,776 --------------------------------------------------------------------------------- Input size (MB): 0.01 Forward/backward pass size (MB): 3.58 Params size (MB): 16.23 Estimated Total Size (MB): 19.82 ---------------------------------------------------------------------------------
{'total_params': 4253864, 'trainable_params': 4210088}
import paddle.vision.transforms as T
transform = T.Compose([
T.Transpose(),
T.Normalize([127.5], [127.5])
])
train_dataset = paddle.vision.datasets.Cifar10(mode="train", backend="cv2",transform=transform)
val_dataset = paddle.vision.datasets.Cifar10(mode="test", backend="cv2",transform=transform)
item 168/41626 [..............................] - ETA: 51s - 1ms/it
Cache file /home/aistudio/.cache/paddle/dataset/cifar/cifar-10-python.tar.gz not found,
downloading https://dataset.bj.bcebos.com/cifar/cifar-10-python.tar.gz Begin to download
item 169/41626 [..............................] - ETA: 51s - 1ms/item
item 170/41626 [..............................] - ETA: 51s - 1ms/item
item 171/41626 [..............................] - ETA: 51s - 1ms/item
item 172/41626 [..............................] - ETA: 51s - 1ms/item
item 173/41626 [..............................] - ETA: 51s - 1ms/item
item 174/41626 [..............................] - ETA: 51s - 1ms/item
item 175/41626 [..............................] - ETA: 51s - 1ms/item
item 176/41626 [..............................] - ETA: 51s - 1ms/item
item 177/41626 [..............................] - ETA: 51s - 1ms/item
item 178/41626 [..............................] - ETA: 51s - 1ms/item
item 179/41626 [..............................] - ETA: 50s - 1ms/item
item 180/41626 [..............................] - ETA: 50s - 1ms/item
item 181/41626 [..............................] - ETA: 51s - 1ms/item
item 182/41626 [..............................] - ETA: 51s - 1ms/item
item 183/41626 [..............................] - ETA: 51s - 1ms/item
item 184/41626 [..............................] - ETA: 50s - 1ms/item
item 185/41626 [..............................] - ETA: 50s - 1ms/item
item 186/41626 [..............................] - ETA: 50s - 1ms/item
item 187/41626 [..............................] - ETA: 50s - 1ms/item
item 188/41626 [..............................] - ETA: 50s - 1ms/item
item 189/41626 [..............................] - ETA: 51s - 1ms/item
item 190/41626 [..............................] - ETA: 50s - 1ms/item
item 191/41626 [..............................] - ETA: 50s - 1ms/item
item 192/41626 [..............................] - ETA: 50s - 1ms/item
item 193/41626 [..............................] - ETA: 50s - 1ms/item
item 194/41626 [..............................] - ETA: 50s - 1ms/item
item 195/41626 [..............................] - ETA: 50s - 1ms/item
item 196/41626 [..............................] - ETA: 49s - 1ms/item
item 197/41626 [..............................] - ETA: 49s - 1ms/item
item 198/41626 [..............................] - ETA: 49s - 1ms/item
item 199/41626 [..............................] - ETA: 49s - 1ms/item
item 200/41626 [..............................] - ETA: 49s - 1ms/item
item 201/41626 [..............................] - ETA: 50s - 1ms/item
item 202/41626 [..............................] - ETA: 50s - 1ms/item
item 203/41626 [..............................] - ETA: 50s - 1ms/item
item 204/41626 [..............................] - ETA: 49s - 1ms/item
item 205/41626 [..............................] - ETA: 49s - 1ms/item
item 206/41626 [..............................] - ETA: 49s - 1ms/item
item 207/41626 [..............................] - ETA: 49s - 1ms/item
item 208/41626 [..............................] - ETA: 49s - 1ms/item
item 209/41626 [..............................] - ETA: 49s - 1ms/item
item 210/41626 [..............................] - ETA: 49s - 1ms/item
item 211/41626 [..............................] - ETA: 49s - 1ms/item
item 212/41626 [..............................] - ETA: 48s - 1ms/item
item 213/41626 [..............................] - ETA: 49s - 1ms/item
item 214/41626 [..............................] - ETA: 49s - 1ms/item
item 215/41626 [..............................] - ETA: 49s - 1ms/item
item 216/41626 [..............................] - ETA: 48s - 1ms/item
item 217/41626 [..............................] - ETA: 48s - 1ms/item
item 218/41626 [..............................] - ETA: 48s - 1ms/item
item 219/41626 [..............................] - ETA: 48s - 1ms/item
item 220/41626 [..............................] - ETA: 48s - 1ms/item
item 221/41626 [..............................] - ETA: 48s - 1ms/item
item 222/41626 [..............................] - ETA: 48s - 1ms/item
item 223/41626 [..............................] - ETA: 48s - 1ms/item
item 224/41626 [..............................] - ETA: 48s - 1ms/item
item 225/41626 [..............................] - ETA: 48s - 1ms/item
item 226/41626 [..............................] - ETA: 48s - 1ms/item
item 227/41626 [..............................] - ETA: 47s - 1ms/item
item 228/41626 [..............................] - ETA: 47s - 1ms/item
item 229/41626 [..............................] - ETA: 48s - 1ms/item
item 230/41626 [..............................] - ETA: 47s - 1ms/item
item 231/41626 [..............................] - ETA: 47s - 1ms/item
item 232/41626 [..............................] - ETA: 47s - 1ms/item
item 233/41626 [..............................] - ETA: 47s - 1ms/item
item 234/41626 [..............................] - ETA: 47s - 1ms/item
item 235/41626 [..............................] - ETA: 47s - 1ms/item
item 236/41626 [..............................] - ETA: 47s - 1ms/item
item 237/41626 [..............................] - ETA: 47s - 1ms/item
item 238/41626 [..............................] - ETA: 47s - 1ms/item
item 239/41626 [..............................] - ETA: 47s - 1ms/item
item 240/41626 [..............................] - ETA: 47s - 1ms/item
item 241/41626 [..............................] - ETA: 48s - 1ms/item
item 242/41626 [..............................] - ETA: 48s - 1ms/item
item 243/41626 [..............................] - ETA: 47s - 1ms/item
item 244/41626 [..............................] - ETA: 47s - 1ms/item
item 245/41626 [..............................] - ETA: 47s - 1ms/item
item 246/41626 [..............................] - ETA: 47s - 1ms/item
item 247/41626 [..............................] - ETA: 47s - 1ms/item
item 248/41626 [..............................] - ETA: 47s - 1ms/item
item 249/41626 [..............................] - ETA: 47s - 1ms/item
item 250/41626 [..............................] - ETA: 47s - 1ms/item
item 251/41626 [..............................] - ETA: 47s - 1ms/item
item 252/41626 [..............................] - ETA: 47s - 1ms/item
item 253/41626 [..............................] - ETA: 47s - 1ms/item
item 254/41626 [..............................] - ETA: 47s - 1ms/item
item 255/41626 [..............................] - ETA: 47s - 1ms/item
item 256/41626 [..............................] - ETA: 47s - 1ms/item
item 257/41626 [..............................] - ETA: 47s - 1ms/item
item 258/41626 [..............................] - ETA: 47s - 1ms/item
item 259/41626 [..............................] - ETA: 47s - 1ms/item
item 260/41626 [..............................] - ETA: 47s - 1ms/item
item 261/41626 [..............................] - ETA: 47s - 1ms/item
item 262/41626 [..............................] - ETA: 47s - 1ms/item
item 263/41626 [..............................] - ETA: 47s - 1ms/item
item 264/41626 [..............................] - ETA: 47s - 1ms/item
item 265/41626 [..............................] - ETA: 47s - 1ms/item
item 266/41626 [..............................] - ETA: 47s - 1ms/item
item 267/41626 [..............................] - ETA: 47s - 1ms/item
item 268/41626 [..............................] - ETA: 47s - 1ms/item
item 269/41626 [..............................] - ETA: 48s - 1ms/item
item 270/41626 [..............................] - ETA: 47s - 1ms/item
item 271/41626 [..............................] - ETA: 47s - 1ms/item
item 272/41626 [..............................] - ETA: 47s - 1ms/item
item 273/41626 [..............................] - ETA: 47s - 1ms/item
item 274/41626 [..............................] - ETA: 47s - 1ms/item
item 275/41626 [..............................] - ETA: 47s - 1ms/item
item 276/41626 [..............................] - ETA: 47s - 1ms/item
item 277/41626 [..............................] - ETA: 47s - 1ms/item
item 278/41626 [..............................] - ETA: 47s - 1ms/item
item 279/41626 [..............................] - ETA: 47s - 1ms/item
item 280/41626 [..............................] - ETA: 47s - 1ms/item
item 281/41626 [..............................] - ETA: 47s - 1ms/item
item 282/41626 [..............................] - ETA: 47s - 1ms/item
item 283/41626 [..............................] - ETA: 47s - 1ms/item
item 284/41626 [..............................] - ETA: 47s - 1ms/item
item 285/41626 [..............................] - ETA: 48s - 1ms/item
item 286/41626 [..............................] - ETA: 48s - 1ms/item
item 287/41626 [..............................] - ETA: 47s - 1ms/item
item 288/41626 [..............................] - ETA: 47s - 1ms/item
item 289/41626 [..............................] - ETA: 47s - 1ms/item
item 290/41626 [..............................] - ETA: 47s - 1ms/item
item 291/41626 [..............................] - ETA: 47s - 1ms/item
item 292/41626 [..............................] - ETA: 47s - 1ms/item
item 293/41626 [..............................] - ETA: 47s - 1ms/item
item 294/41626 [..............................] - ETA: 47s - 1ms/item
item 295/41626 [..............................] - ETA: 47s - 1ms/item
item 296/41626 [..............................] - ETA: 47s - 1ms/item
item 297/41626 [..............................] - ETA: 47s - 1ms/item
item 298/41626 [..............................] - ETA: 47s - 1ms/item
item 299/41626 [..............................] - ETA: 47s - 1ms/item
item 300/41626 [..............................] - ETA: 47s - 1ms/item
item 301/41626 [..............................] - ETA: 47s - 1ms/item
item 302/41626 [..............................] - ETA: 47s - 1ms/item
item 303/41626 [..............................] - ETA: 46s - 1ms/item
item 304/41626 [..............................] - ETA: 46s - 1ms/item
item 305/41626 [..............................] - ETA: 46s - 1ms/item
item 306/41626 [..............................] - ETA: 46s - 1ms/item
item 307/41626 [..............................] - ETA: 46s - 1ms/item
item 308/41626 [..............................] - ETA: 46s - 1ms/item
item 309/41626 [..............................] - ETA: 46s - 1ms/item
item 310/41626 [..............................] - ETA: 46s - 1ms/item
item 311/41626 [..............................] - ETA: 46s - 1ms/item
item 312/41626 [..............................] - ETA: 46s - 1ms/item
item 313/41626 [..............................] - ETA: 46s - 1ms/item
item 314/41626 [..............................] - ETA: 46s - 1ms/item
item 315/41626 [..............................] - ETA: 46s - 1ms/item
item 316/41626 [..............................] - ETA: 46s - 1ms/item
item 317/41626 [..............................] - ETA: 46s - 1ms/item
item 318/41626 [..............................] - ETA: 46s - 1ms/item
item 319/41626 [..............................] - ETA: 46s - 1ms/item
item 320/41626 [..............................] - ETA: 46s - 1ms/item
item 321/41626 [..............................] - ETA: 46s - 1ms/item
item 322/41626 [..............................] - ETA: 46s - 1ms/item
item 323/41626 [..............................] - ETA: 46s - 1ms/item
item 324/41626 [..............................] - ETA: 46s - 1ms/item
item 325/41626 [..............................] - ETA: 46s - 1ms/item
item 326/41626 [..............................] - ETA: 46s - 1ms/item
item 327/41626 [..............................] - ETA: 46s - 1ms/item
item 328/41626 [..............................] - ETA: 46s - 1ms/item
item 329/41626 [..............................] - ETA: 46s - 1ms/item
item 330/41626 [..............................] - ETA: 46s - 1ms/item
item 331/41626 [..............................] - ETA: 46s - 1ms/item
item 332/41626 [..............................] - ETA: 46s - 1ms/item
item 333/41626 [..............................] - ETA: 46s - 1ms/item
item 334/41626 [..............................] - ETA: 46s - 1ms/item
item 335/41626 [..............................] - ETA: 46s - 1ms/item
item 336/41626 [..............................] - ETA: 46s - 1ms/item
item 337/41626 [..............................] - ETA: 46s - 1ms/item
item 338/41626 [..............................] - ETA: 46s - 1ms/item
item 339/41626 [..............................] - ETA: 46s - 1ms/item
item 340/41626 [..............................] - ETA: 45s - 1ms/item
item 341/41626 [..............................] - ETA: 45s - 1ms/item
item 342/41626 [..............................] - ETA: 45s - 1ms/item
item 343/41626 [..............................] - ETA: 45s - 1ms/item
item 344/41626 [..............................] - ETA: 45s - 1ms/item
item 345/41626 [..............................] - ETA: 45s - 1ms/item
item 346/41626 [..............................] - ETA: 45s - 1ms/item
item 347/41626 [..............................] - ETA: 45s - 1ms/item
item 348/41626 [..............................] - ETA: 45s - 1ms/item
item 349/41626 [..............................] - ETA: 45s - 1ms/item
item 350/41626 [..............................] - ETA: 45s - 1ms/item
item 351/41626 [..............................] - ETA: 45s - 1ms/item
item 352/41626 [..............................] - ETA: 45s - 1ms/item
item 353/41626 [..............................] - ETA: 45s - 1ms/item
item 354/41626 [..............................] - ETA: 45s - 1ms/item
item 355/41626 [..............................] - ETA: 45s - 1ms/item
item 356/41626 [..............................] - ETA: 45s - 1ms/item
item 357/41626 [..............................] - ETA: 45s - 1ms/item
item 358/41626 [..............................] - ETA: 45s - 1ms/item
item 359/41626 [..............................] - ETA: 44s - 1ms/item
item 360/41626 [..............................] - ETA: 44s - 1ms/item
item 361/41626 [..............................] - ETA: 44s - 1ms/item
item 362/41626 [..............................] - ETA: 44s - 1ms/item
item 363/41626 [..............................] - ETA: 44s - 1ms/item
item 364/41626 [..............................] - ETA: 44s - 1ms/item
item 365/41626 [..............................] - ETA: 44s - 1ms/item
item 366/41626 [..............................] - ETA: 44s - 1ms/item
item 367/41626 [..............................] - ETA: 44s - 1ms/item
item 368/41626 [..............................] - ETA: 44s - 1ms/item
item 369/41626 [..............................] - ETA: 44s - 1ms/item
item 596/41626 [..............................] - ETA: 38s - 941us/i item 2876/41626 [=>............................] - ETA: 28s - 748us/it
IOPub message rate exceeded. The notebook server will temporarily stop sending output to the client in order to avoid crashing it. To change this limit, set the config variable `--NotebookApp.iopub_msg_rate_limit`. Current values: NotebookApp.iopub_msg_rate_limit=1000.0 (msgs/sec) NotebookApp.rate_limit_window=3.0 (secs)
item 4245/41626 [==>...........................] - ETA: 26s - 722us/i item 6880/41626 [===>..........................] - ETA: 25s - 724us/ite
IOPub message rate exceeded. The notebook server will temporarily stop sending output to the client in order to avoid crashing it. To change this limit, set the config variable `--NotebookApp.iopub_msg_rate_limit`. Current values: NotebookApp.iopub_msg_rate_limit=1000.0 (msgs/sec) NotebookApp.rate_limit_window=3.0 (secs)
item 8013/41626 [====>.........................] - ETA: 25s - 748us/i item 10647/41626 [======>.......................] - ETA: 24s - 787us/it
IOPub message rate exceeded. The notebook server will temporarily stop sending output to the client in order to avoid crashing it. To change this limit, set the config variable `--NotebookApp.iopub_msg_rate_limit`. Current values: NotebookApp.iopub_msg_rate_limit=1000.0 (msgs/sec) NotebookApp.rate_limit_window=3.0 (secs)
item 11791/41626 [=======>......................] - ETA: 23s - 790us/it item 14536/41626 [=========>....................] - ETA: 21s - 788us/item
IOPub message rate exceeded. The notebook server will temporarily stop sending output to the client in order to avoid crashing it. To change this limit, set the config variable `--NotebookApp.iopub_msg_rate_limit`. Current values: NotebookApp.iopub_msg_rate_limit=1000.0 (msgs/sec) NotebookApp.rate_limit_window=3.0 (secs)
item 15726/41626 [==========>...................] - ETA: 20s - 786us/it item 18533/41626 [============>.................] - ETA: 17s - 773us/it
IOPub message rate exceeded. The notebook server will temporarily stop sending output to the client in order to avoid crashing it. To change this limit, set the config variable `--NotebookApp.iopub_msg_rate_limit`. Current values: NotebookApp.iopub_msg_rate_limit=1000.0 (msgs/sec) NotebookApp.rate_limit_window=3.0 (secs)
item 19586/41626 [=============>................] - ETA: 17s - 775us/it item 22148/41626 [==============>...............] - ETA: 15s - 782us/ite
IOPub message rate exceeded. The notebook server will temporarily stop sending output to the client in order to avoid crashing it. To change this limit, set the config variable `--NotebookApp.iopub_msg_rate_limit`. Current values: NotebookApp.iopub_msg_rate_limit=1000.0 (msgs/sec) NotebookApp.rate_limit_window=3.0 (secs)
item 23742/41626 [================>.............] - ETA: 13s - 777us/i item 25988/41626 [=================>............] - ETA: 12s - 784us/ite
IOPub message rate exceeded. The notebook server will temporarily stop sending output to the client in order to avoid crashing it. To change this limit, set the config variable `--NotebookApp.iopub_msg_rate_limit`. Current values: NotebookApp.iopub_msg_rate_limit=1000.0 (msgs/sec) NotebookApp.rate_limit_window=3.0 (secs)
item 27110/41626 [==================>...........] - ETA: 11s - 786us/i item 29759/41626 [====================>.........] - ETA: 9s - 788us/it
IOPub message rate exceeded. The notebook server will temporarily stop sending output to the client in order to avoid crashing it. To change this limit, set the config variable `--NotebookApp.iopub_msg_rate_limit`. Current values: NotebookApp.iopub_msg_rate_limit=1000.0 (msgs/sec) NotebookApp.rate_limit_window=3.0 (secs)
item 31110/41626 [=====================>........] - ETA: 8s - 785us/i item 33672/41626 [=======================>......] - ETA: 6s - 783us/it
IOPub message rate exceeded. The notebook server will temporarily stop sending output to the client in order to avoid crashing it. To change this limit, set the config variable `--NotebookApp.iopub_msg_rate_limit`. Current values: NotebookApp.iopub_msg_rate_limit=1000.0 (msgs/sec) NotebookApp.rate_limit_window=3.0 (secs)
item 34816/41626 [========================>.....] - ETA: 5s - 783us/it item 37603/41626 [==========================>...] - ETA: 3s - 800us/it
IOPub message rate exceeded. The notebook server will temporarily stop sending output to the client in order to avoid crashing it. To change this limit, set the config variable `--NotebookApp.iopub_msg_rate_limit`. Current values: NotebookApp.iopub_msg_rate_limit=1000.0 (msgs/sec) NotebookApp.rate_limit_window=3.0 (secs)
item 38788/41626 [==========================>...] - ETA: 2s - 801us/it item 41346/41626 [============================>.] - ETA: 0s - 804us/it
IOPub message rate exceeded. The notebook server will temporarily stop sending output to the client in order to avoid crashing it. To change this limit, set the config variable `--NotebookApp.iopub_msg_rate_limit`. Current values: NotebookApp.iopub_msg_rate_limit=1000.0 (msgs/sec) NotebookApp.rate_limit_window=3.0 (secs)
paddle.vision.transforms下封装了很多预处理图片数据的函数,供用户快速完成数据处理。其中,Compose()函数可以将多个操作组合起来,包括图像格式的变换(Transpose()),和像素值的标准化(Normalize())。Transpose()的目标格式可以通过参数order指定,默认值是(2, 0, 1),代表多数原始图片格式是HWC(Hight, Width, Channel)的顺序,转换成神经网络使用(Channel,Hight, Width)模式的输入张量。
我们可以通过以下代码查看训练集和测试集的样本数量,并尝试取出训练集中的第一个样本,观察其图片的shape和对应的label。
from __future__ import print_function
print(f'train samples count: {len(train_dataset)}')
print(f'val samples count: {len(val_dataset)}') for data in train_dataset:
print(f'image shape: {data[0].shape}; label: {data[1]}') break
train samples count: 50000 val samples count: 10000 image shape: (3, 32, 32); label: 6
from paddle.static import InputSpec as Input
optimizer = paddle.optimizer.Momentum(
learning_rate=0.1,
parameters=net.parameters())
inputs = [Input([None, 3, 32, 32], 'float32', name='image')]
labels = [Input([None], 'int64', name='label')]
model = paddle.Model(net, inputs, labels)
model.prepare(
optimizer,
paddle.nn.CrossEntropyLoss(),
paddle.metric.Accuracy(topk=(1, 5)))
以上代码声明了用于训练的model对象,接下来可以调用model的fit接口和evaluate接口分别进行训练和评估:
model.fit(train_dataset, epochs=2, batch_size=128, verbose=1)
result = model.evaluate(val_dataset,batch_size=128, log_freq=10)
print(result)
The loss value printed in the log is the current step, and the metric is the average value of previous steps. Epoch 1/2
/opt/conda/envs/python35-paddle120-env/lib/python3.7/site-packages/paddle/fluid/layers/utils.py:
77: DeprecationWarning: Using or importing the ABCs from 'collections' instead of from 'collect
ions.abc' is deprecated, and in 3.8 it will stop working return (isinstance(seq, collections.Sequence) and /opt/conda/envs/python35-paddle120-env/lib/python3.7/site-packages/paddle/nn/layer/norm.py:654:
UserWarning: When training, we now always track global mean and variance. "When training, we now always track global mean and variance.")
step 391/391 [==============================] - loss: 1.7519 - acc_top1: 0.3342 - acc_top5: 0.8270 - 35ms/step Epoch 2/2 step 391/391 [==============================] - loss: 1.3786 - acc_top1: 0.4630 - acc_top5: 0.9148 - 35ms/step Eval begin... step 10/79 - loss: 1.5248 - acc_top1: 0.4891 - acc_top5: 0.9234 - 20ms/step step 20/79 - loss: 1.6803 - acc_top1: 0.4801 - acc_top5: 0.9133 - 19ms/step step 30/79 - loss: 1.3865 - acc_top1: 0.4833 - acc_top5: 0.9161 - 19ms/step step 40/79 - loss: 1.4153 - acc_top1: 0.4820 - acc_top5: 0.9176 - 20ms/step step 50/79 - loss: 1.3966 - acc_top1: 0.4792 - acc_top5: 0.9191 - 19ms/step step 60/79 - loss: 1.5109 - acc_top1: 0.4806 - acc_top5: 0.9187 - 19ms/step step 70/79 - loss: 1.4490 - acc_top1: 0.4765 - acc_top5: 0.9190 - 19ms/step step 79/79 - loss: 1.5520 - acc_top1: 0.4757 - acc_top5: 0.9200 - 19ms/step Eval samples: 10000 {'loss': [1.5520492], 'acc_top1': 0.4757, 'acc_top5': 0.92}
4. 剪裁
本节内容分为两部分:卷积层重要性分析和Filters剪裁,其中『卷积层重要性分析』也可以被称作『卷积层敏感度分析』,我们定义越重要的卷积层越敏感。敏感度的理论计算过程如下图所示:第一层卷积操作有四个卷积核,首先计算每个卷积核参数的L1_norm值,即所有参数的绝对值之和。之后按照每个卷积的L1_norm值排序,先去掉L1_norm值最小的(即图中L1_norm=1的卷积核),测试模型的效果变化,再去掉次小的(即图中L1_norm=1.2的卷积核),测试模型的效果变换,以此类推。观察每次裁剪的模型效果曲线绘图,那些裁剪后模型效果衰减不显著的卷积核会被删除。因此,敏感度通俗的理解就是每个卷积核对最终预测结果的贡献度或者有效性,那些对最终结果影响不大的部分会被裁掉。
图:卷积核裁剪的计算逻辑
PaddleSlim提供了工具类Pruner来进行重要性分析和剪裁操作,不同的Pruner的子类对应不同的分析和剪裁策略,本示例以L1NormFilterPruner为例说明。首先我们声明一个L1NormFilterPruner对象,如下所示:
from paddleslim.dygraph import L1NormFilterPruner
pruner = L1NormFilterPruner(net, [1, 3, 224, 224])
[05-06 16:03:37 MainThread @utils.py:79] WRN paddlepaddle version: 2.3.0-rc0. The dynamic
graph version of PARL is under development, not fully tested and supported
/opt/conda/envs/python35-paddle120-env/lib/python3.7/site-packages/paddleslim/core/graph_wrapper
.py:20: DeprecationWarning: Using or importing the ABCs from 'collections' instead of from '
collections.abc' is deprecated, and in 3.8 it will stop working from collections import Iterable /opt/conda/envs/python35-paddle120-env/lib/python3.7/site-packages/parl/remote/communication.py:38:
DeprecationWarning: 'pyarrow.default_serialization_context' is deprecated as of 2.0.0 and will
be removed in a future version. Use pickle or the pyarrow IPC functionality instead. context = pyarrow.default_serialization_context() /opt/conda/envs/python35-paddle120-env/lib/python3.7/site-packages/matplotlib/__init__.py:107:
DeprecationWarning: Using or importing the ABCs from 'collections' instead of from 'collections.abc'
is deprecated, and in 3.8 it will stop working from collections import MutableMapping /opt/conda/envs/python35-paddle120-env/lib/python3.7/site-packages/matplotlib/rcsetup.py:20:
DeprecationWarning: Using or importing the ABCs from 'collections' instead of from 'collections.abc'
is deprecated, and in 3.8 it will stop working from collections import Iterable, Mapping /opt/conda/envs/python35-paddle120-env/lib/python3.7/site-packages/matplotlib/colors.py:53:
DeprecationWarning: Using or importing the ABCs from 'collections' instead of from 'collections.abc'
is deprecated, and in 3.8 it will stop working from collections import Sized 2022-05-06 16:03:39,140-WARNING: type object 'QuantizationTransformPass' has no
attribute '_supported_quantizable_op_type' 2022-05-06 16:03:39,141-WARNING: If you want to use training-aware and post-training quantization,
please use Paddle >= 1.8.4 or develop version 2022-05-06 16:03:39,689-INFO: No walker for operator: matmul_v2 2022-05-06 16:03:39,691-INFO: Found 14 groups.
如果本地文件系统已有一个存储敏感度信息(见4.1节)的文件,声明L1NormFilterPruner对象时,可以通过指定sen_file选项加载计算好的敏感度信息,如下:
#pruner = L1NormFilterPruner(net, [1, 3, 224, 224]), sen_file="./sen.pickle")
def eval_fn(): result = model.evaluate(
val_dataset,
batch_size=128) return result['acc_top1']
pruner.sensitive(eval_func=eval_fn, sen_file="./sen.pickle")
2022-05-06 16:03:39,702-INFO: Load status from ./sen.pickle
{'conv2d_0.w_0': {0.1: nan, 0.2: 0.0, 0.3: 0.7603068072866731, 0.4: 0.7603068072866731,
0.5: 0.7603068072866731, 0.6: 0.7603068072866731, 0.7: 0.6743527508090615, 0.8: 0.
7926779935275081, 0.9: 0.759911003236246}, 'conv2d_2.w_0': {0.1: 0.006877022653721711,
0.2: 0.019619741100323596, 0.3: 0.28580097087378636, 0.4: 0.42738673139158573, 0.5:
0.49716828478964403, 0.6: 0.5641181229773463, 0.7: 0.7006472491909386, 0.8: 0.7495954692556634,
0.9: 0.7597087378640777}, 'conv2d_4.w_0': {0.1: -0.005056634304207124, 0.2: 0.019215210355987073,
0.3: 0.016585760517799322, 0.4: 0.06674757281553403, 0.5: 0.12216828478964403, 0.6: 0.
4678398058252427, 0.7: 0.5833333333333333, 0.8: 0.6213592233009709, 0.9: 0.6858818770226537},
'conv2d_6.w_0': {0.1: 0.008697411003236299, 0.2: 0.03013754045307448, 0.3: 0.06492718446601946,
0.4: 0.13288834951456308, 0.5: 0.20165857605177995, 0.6: 0.3836974110032362, 0.7: 0.6296521035598706,
0.8: 0.7497977346278317, 0.9: 0.7686084142394822}, 'conv2d_8.w_0': {0.1: 0.003843042071197437,
0.2: 0.020833333333333398, 0.3: 0.012742718446601999, 0.4: 0.03135113268608417, 0.5: 0.08029935275080909,
0.6: 0.12540453074433658, 0.7: 0.2332119741100324, 0.8: 0.2866100323624595, 0.9: 0.5378236245954693},
'conv2d_10.w_0': {0.1: 0.015169902912621373, 0.2: 0.03863268608414241, 0.3: 0.06553398058252424,
0.4: 0.13127022653721684, 0.5: 0.1783980582524272, 0.6: 0.2684061488673139, 0.7: 0.32625404530744334,
0.8: 0.568163430420712, 0.9: 0.6401699029126213}, 'conv2d_12.w_0': {0.1: 0.0012135922330096874,
0.2: 0.019619741100323596, 0.3: 0.09567152103559873, 0.4: 0.1563511326860841, 0.5: 0.21318770226537215,
0.6: 0.3042071197411004, 0.7: 0.4712783171521035, 0.8: 0.662621359223301, 0.9: 0.7578883495145632},
'conv2d_14.w_0': {0.1: 0.020024271844660234, 0.2: 0.09769417475728157, 0.3: 0.19073624595469255,
0.4: 0.2653721682847896, 0.5: 0.334546925566343, 0.6: 0.42556634304207125, 0.7: 0.48179611650485443,
0.8: 0.5232605177993528, 0.9: 0.5455097087378641}, 'conv2d_16.w_0': {0.1: 0.054409385113268566,
0.2: 0.19700647249190936, 0.3: 0.29813915857605183, 0.4: 0.39745145631067963, 0.5: 0.46480582524271846,
0.6: 0.5358009708737864, 0.7: 0.5845469255663431, 0.8: 0.6699029126213593, 0.9: 0.7870145631067961},
'conv2d_18.w_0': {0.1: 0.031148867313915907, 0.2: 0.13187702265372164, 0.3: 0.255663430420712,
0.4: 0.377831715210356, 0.5: 0.4783576051779935, 0.6: 0.5744336569579288, 0.7: 0.6102346278317151,
0.8: 0.6992313915857605, 0.9: 0.7843851132686085}, 'conv2d_20.w_0': {0.1: 0.014765372168284847,
0.2: 0.046116504854368905, 0.3: 0.13167475728155337, 0.4: 0.31599426582019247, 0.5: 0.4925250870366578,
0.6: 0.5820192504607823, 0.7: 0.6135572394020069, 0.8: 0.6194962113454843, 0.9: 0.6293262338726193},
'conv2d_22.w_0': {0.1: 0.00634855621544131, 0.2: 0.016588163014540233, 0.3: 0.02621339340569329,
0.4: 0.05140282613147657, 0.5: 0.10587753430268282, 0.6: 0.18267458529592465, 0.7: 0.2883473274626255,
0.8: 0.4386647552733975, 0.9: 0.6688511161171411}, 'conv2d_24.w_0': {0.1: 0.0, 0.2:
0.0010239606799098923, 0.3: 0.01392586524677458, 0.4: 0.017202539422486215, 0.5: 0.010239606799098924,
0.6: 0.02621339340569329, 0.7: 0.09174687691992628, 0.8: 0.1464263772271145, 0.9: 0.3704689739913987},
'conv2d_26.w_0': {0.1: -0.0006143764079458672, 0.2: 0.0016383370878558733, 0.3: 0.001228752815891848,
0.4: 0.003686258447675658, 0.5: 0.012287528158918709, 0.6: 0.030514028261314816, 0.7: 0
.05529387671513419, 0.8: 0.10915420847839445, 0.9: 0.21216465287732955}}
上述代码执行完毕后,敏感度信息会存放在pruner对象中,可以通过以下方式查看敏感度信息内容:
print(pruner.sensitive())
{'conv2d_0.w_0': {0.1: nan, 0.2: 0.0, 0.3: 0.7603068072866731, 0.4: 0.7603068072866731, 0.5:
0.7603068072866731, 0.6: 0.7603068072866731, 0.7: 0.6743527508090615, 0.8: 0.7926779935275081,
0.9: 0.759911003236246}, 'conv2d_2.w_0': {0.1: 0.006877022653721711, 0.2: 0.019619741100323596,
0.3: 0.28580097087378636, 0.4: 0.42738673139158573, 0.5: 0.49716828478964403, 0.6: 0.56411812297
73463, 0.7: 0.7006472491909386, 0.8: 0.7495954692556634, 0.9: 0.7597087378640777}, 'conv2d_4.w_0':
{0.1: -0.005056634304207124, 0.2: 0.019215210355987073, 0.3: 0.016585760517799322, 0.4: 0.0667475
7281553403, 0.5: 0.12216828478964403, 0.6: 0.4678398058252427, 0.7: 0.5833333333333333, 0.8: 0.62
13592233009709, 0.9: 0.6858818770226537}, 'conv2d_6.w_0': {0.1: 0.008697411003236299, 0.2: 0.03013
754045307448, 0.3: 0.06492718446601946, 0.4: 0.13288834951456308, 0.5: 0.20165857605177995, 0.6: 0
.3836974110032362, 0.7: 0.6296521035598706, 0.8: 0.7497977346278317, 0.9: 0.7686084142394822}, 'co
nv2d_8.w_0': {0.1: 0.003843042071197437, 0.2: 0.020833333333333398, 0.3: 0.012742718446601999, 0.4:
0.03135113268608417, 0.5: 0.08029935275080909, 0.6: 0.12540453074433658, 0.7: 0.2332119741100324, 0.8:
0.2866100323624595, 0.9: 0.5378236245954693}, 'conv2d_10.w_0': {0.1: 0.015169902912621373, 0.2:
0.03863268608414241, 0.3: 0.06553398058252424, 0.4: 0.13127022653721684, 0.5: 0.1783980582524272,
0.6: 0.2684061488673139, 0.7: 0.32625404530744334, 0.8: 0.568163430420712, 0.9: 0.6401699029126213},
'conv2d_12.w_0': {0.1: 0.0012135922330096874, 0.2: 0.019619741100323596, 0.3: 0.09567152103559873,
0.4: 0.1563511326860841, 0.5: 0.21318770226537215, 0.6: 0.3042071197411004, 0.7: 0.4712783171521035,
0.8: 0.662621359223301, 0.9: 0.7578883495145632}, 'conv2d_14.w_0': {0.1: 0.020024271844660234,
0.2: 0.09769417475728157, 0.3: 0.19073624595469255, 0.4: 0.2653721682847896, 0.5: 0.334546925566343,
0.6: 0.42556634304207125, 0.7: 0.48179611650485443, 0.8: 0.5232605177993528, 0.9: 0.5455097087378641}
, 'conv2d_16.w_0': {0.1: 0.054409385113268566, 0.2: 0.19700647249190936, 0.3: 0.29813915857605183,
0.4: 0.39745145631067963, 0.5: 0.46480582524271846, 0.6: 0.5358009708737864, 0.7: 0.5845469255663431,
0.8: 0.6699029126213593, 0.9: 0.7870145631067961}, 'conv2d_18.w_0': {0.1: 0.031148867313915907, 0.2:
0.13187702265372164, 0.3: 0.255663430420712, 0.4: 0.377831715210356, 0.5: 0.4783576051779935, 0.6: 0
.5744336569579288, 0.7: 0.6102346278317151, 0.8: 0.6992313915857605, 0.9: 0.7843851132686085},
'conv2d_20.w_0': {0.1: 0.014765372168284847, 0.2: 0.046116504854368905, 0.3: 0.13167475728155337,
0.4: 0.31599426582019247, 0.5: 0.4925250870366578, 0.6: 0.5820192504607823, 0.7: 0.6135572394020069,
0.8: 0.6194962113454843, 0.9: 0.6293262338726193}, 'conv2d_22.w_0': {0.1: 0.00634855621544131, 0.2
: 0.016588163014540233, 0.3: 0.02621339340569329, 0.4: 0.05140282613147657, 0.5: 0.10587753430268282,
0.6: 0.18267458529592465, 0.7: 0.2883473274626255, 0.8: 0.4386647552733975, 0.9: 0.6688511161171411},
'conv2d_24.w_0': {0.1: 0.0, 0.2: 0.0010239606799098923, 0.3: 0.01392586524677458, 0.4:
0.017202539422486215, 0.5: 0.010239606799098924, 0.6: 0.02621339340569329, 0.7: 0.09174687691992628,
0.8: 0.1464263772271145, 0.9: 0.3704689739913987}, 'conv2d_26.w_0': {0.1: -0.0006143764079458672, 0.2:
0.0016383370878558733, 0.3: 0.001228752815891848, 0.4: 0.003686258447675658, 0.5: 0.012287528158918709,
0.6: 0.030514028261314816, 0.7: 0.05529387671513419, 0.8: 0.10915420847839445, 0.9: 0.21216465287732955}}
pruner.sensitive()返回的是一个存储敏感度信息的字典sensitivities(dict),示例如下:
{“weight_0”: {0.1: 0.22, 0.2: 0.33 }, “weight_1”: {0.1: 0.21, 0.2: 0.4 } } 其中,weight_0 是卷积层权重变量的名称, sensitivities[‘weight_0’] 是一个字典, key是用 float 类型数值表示的剪裁率,value是对应剪裁率下整个模型的精度损失比例。以上述案例来看,对于卷积核weight_0来说,裁剪比例从0.1升高到0.2的话,精度损失会由-22%提升到-33%。
from paddleslim.analysis import dygraph_flops
flops = dygraph_flops(net, [1, 3, 32, 32])
print(f"FLOPs before pruning: {flops}")
FLOPs before pruning: 11792896.0
执行剪裁操作,期望跳过最后一层卷积层并剪掉40%的FLOPs,skip_vars参数可以指定不期望裁剪的参数结构。
plan = pruner.sensitive_prune(0.4, skip_vars=["conv2d_26.w_0"])
flops = dygraph_flops(net, [1, 3, 32, 32])
print(f"FLOPs after pruning: {flops}")
print(f"Pruned FLOPs: {round(plan.pruned_flops*100, 2)}%")
FLOPs after pruning: 7077099.0 Pruned FLOPs: 39.99%
通常,剪裁之后,模型的精度会大幅下降。如下所示,在测试集上重新评估精度,精度大幅下降:
result = model.evaluate(val_dataset,batch_size=128, log_freq=10)
print(f"before fine-tuning: {result}")
Eval begin... step 10/79 - loss: 2.0944 - acc_top1: 0.2164 - acc_top5: 0.7289 - 27ms/step step 20/79 - loss: 2.1410 - acc_top1: 0.2156 - acc_top5: 0.7051 - 24ms/step step 30/79 - loss: 2.1013 - acc_top1: 0.2128 - acc_top5: 0.7023 - 23ms/step step 40/79 - loss: 2.1410 - acc_top1: 0.2096 - acc_top5: 0.7035 - 23ms/step step 50/79 - loss: 2.0900 - acc_top1: 0.2048 - acc_top5: 0.7037 - 22ms/step step 60/79 - loss: 2.0988 - acc_top1: 0.2033 - acc_top5: 0.7029 - 22ms/step step 70/79 - loss: 2.1262 - acc_top1: 0.2059 - acc_top5: 0.7060 - 22ms/step step 79/79 - loss: 1.9119 - acc_top1: 0.2071 - acc_top5: 0.7073 - 22ms/step Eval samples: 10000 before fine-tuning: {'loss': [1.911865], 'acc_top1': 0.2071, 'acc_top5': 0.7073}
因此,需要对剪裁后的模型重新训练, 从而提升模型的精度,精度的提升取决于模型的要求。我们再训练之后在测试集上再次测试精度,会发现精度提升如下:
optimizer = paddle.optimizer.Momentum(
learning_rate=0.1,
parameters=net.parameters())
model.prepare(
optimizer,
paddle.nn.CrossEntropyLoss(),
paddle.metric.Accuracy(topk=(1, 5)))
model.fit(train_dataset, epochs=2, batch_size=128, verbose=1)
result = model.evaluate(val_dataset,batch_size=128, log_freq=10)
print(f"after fine-tuning: {result}")
The loss value printed in the log is the current step, and the metric is the average value of previous steps. Epoch 1/2
/opt/conda/envs/python35-paddle120-env/lib/python3.7/site-packages/paddle/nn/layer/norm.py:654:
UserWarning: When training, we now always track global mean and variance. "When training, we now always track global mean and variance.")
step 391/391 [==============================] - loss: 1.4295 - acc_top1: 0.4880 - acc_top5: 0.9197 - 38ms/step Epoch 2/2 step 391/391 [==============================] - loss: 1.2351 - acc_top1: 0.5386 - acc_top5: 0.9369 - 38ms/step Eval begin... step 10/79 - loss: 1.3599 - acc_top1: 0.5398 - acc_top5: 0.9422 - 25ms/step step 20/79 - loss: 1.5895 - acc_top1: 0.5273 - acc_top5: 0.9340 - 23ms/step step 30/79 - loss: 1.2653 - acc_top1: 0.5336 - acc_top5: 0.9307 - 22ms/step step 40/79 - loss: 1.2381 - acc_top1: 0.5334 - acc_top5: 0.9324 - 21ms/step step 50/79 - loss: 1.2706 - acc_top1: 0.5306 - acc_top5: 0.9325 - 21ms/step step 60/79 - loss: 1.3466 - acc_top1: 0.5310 - acc_top5: 0.9345 - 21ms/step step 70/79 - loss: 1.3419 - acc_top1: 0.5292 - acc_top5: 0.9350 - 20ms/step step 79/79 - loss: 1.3053 - acc_top1: 0.5286 - acc_top5: 0.9357 - 20ms/step Eval samples: 10000 after fine-tuning: {'loss': [1.3053463], 'acc_top1': 0.5286, 'acc_top5': 0.9357}
经过重新训练,精度有所提升,最后看下剪裁后模型的结构信息,如下:
paddle.summary(net, (1, 3, 32, 32))
--------------------------------------------------------------------------------- Layer (type) Input Shape Output Shape Param # ================================================================================= Conv2D-1 [[1, 3, 32, 32]] [1, 25, 16, 16] 675 BatchNorm2D-1 [[1, 25, 16, 16]] [1, 25, 16, 16] 100 ReLU-1 [[1, 25, 16, 16]] [1, 25, 16, 16] 0 ConvBNLayer-1 [[1, 3, 32, 32]] [1, 25, 16, 16] 0 Conv2D-2 [[1, 25, 16, 16]] [1, 25, 16, 16] 225 BatchNorm2D-2 [[1, 25, 16, 16]] [1, 25, 16, 16] 100 ReLU-2 [[1, 25, 16, 16]] [1, 25, 16, 16] 0 ConvBNLayer-2 [[1, 25, 16, 16]] [1, 25, 16, 16] 0 Conv2D-3 [[1, 25, 16, 16]] [1, 51, 16, 16] 1,275 BatchNorm2D-3 [[1, 51, 16, 16]] [1, 51, 16, 16] 204 ReLU-3 [[1, 51, 16, 16]] [1, 51, 16, 16] 0 ConvBNLayer-3 [[1, 25, 16, 16]] [1, 51, 16, 16] 0 DepthwiseSeparable-1 [[1, 25, 16, 16]] [1, 51, 16, 16] 0 Conv2D-4 [[1, 51, 16, 16]] [1, 51, 8, 8] 459 BatchNorm2D-4 [[1, 51, 8, 8]] [1, 51, 8, 8] 204 ReLU-4 [[1, 51, 8, 8]] [1, 51, 8, 8] 0 ConvBNLayer-4 [[1, 51, 16, 16]] [1, 51, 8, 8] 0 Conv2D-5 [[1, 51, 8, 8]] [1, 83, 8, 8] 4,233 BatchNorm2D-5 [[1, 83, 8, 8]] [1, 83, 8, 8] 332 ReLU-5 [[1, 83, 8, 8]] [1, 83, 8, 8] 0 ConvBNLayer-5 [[1, 51, 8, 8]] [1, 83, 8, 8] 0 DepthwiseSeparable-2 [[1, 51, 16, 16]] [1, 83, 8, 8] 0 Conv2D-6 [[1, 83, 8, 8]] [1, 83, 8, 8] 747 BatchNorm2D-6 [[1, 83, 8, 8]] [1, 83, 8, 8] 332 ReLU-6 [[1, 83, 8, 8]] [1, 83, 8, 8] 0 ConvBNLayer-6 [[1, 83, 8, 8]] [1, 83, 8, 8] 0 Conv2D-7 [[1, 83, 8, 8]] [1, 98, 8, 8] 8,134 BatchNorm2D-7 [[1, 98, 8, 8]] [1, 98, 8, 8] 392 ReLU-7 [[1, 98, 8, 8]] [1, 98, 8, 8] 0 ConvBNLayer-7 [[1, 83, 8, 8]] [1, 98, 8, 8] 0 DepthwiseSeparable-3 [[1, 83, 8, 8]] [1, 98, 8, 8] 0 Conv2D-8 [[1, 98, 8, 8]] [1, 98, 4, 4] 882 BatchNorm2D-8 [[1, 98, 4, 4]] [1, 98, 4, 4] 392 ReLU-8 [[1, 98, 4, 4]] [1, 98, 4, 4] 0 ConvBNLayer-8 [[1, 98, 8, 8]] [1, 98, 4, 4] 0 Conv2D-9 [[1, 98, 4, 4]] [1, 147, 4, 4] 14,406 BatchNorm2D-9 [[1, 147, 4, 4]] [1, 147, 4, 4] 588 ReLU-9 [[1, 147, 4, 4]] [1, 147, 4, 4] 0 ConvBNLayer-9 [[1, 98, 4, 4]] [1, 147, 4, 4] 0 DepthwiseSeparable-4 [[1, 98, 8, 8]] [1, 147, 4, 4] 0 Conv2D-10 [[1, 147, 4, 4]] [1, 147, 4, 4] 1,323 BatchNorm2D-10 [[1, 147, 4, 4]] [1, 147, 4, 4] 588 ReLU-10 [[1, 147, 4, 4]] [1, 147, 4, 4] 0 ConvBNLayer-10 [[1, 147, 4, 4]] [1, 147, 4, 4] 0 Conv2D-11 [[1, 147, 4, 4]] [1, 200, 4, 4] 29,400 BatchNorm2D-11 [[1, 200, 4, 4]] [1, 200, 4, 4] 800 ReLU-11 [[1, 200, 4, 4]] [1, 200, 4, 4] 0 ConvBNLayer-11 [[1, 147, 4, 4]] [1, 200, 4, 4] 0 DepthwiseSeparable-5 [[1, 147, 4, 4]] [1, 200, 4, 4] 0 Conv2D-12 [[1, 200, 4, 4]] [1, 200, 2, 2] 1,800 BatchNorm2D-12 [[1, 200, 2, 2]] [1, 200, 2, 2] 800 ReLU-12 [[1, 200, 2, 2]] [1, 200, 2, 2] 0 ConvBNLayer-12 [[1, 200, 4, 4]] [1, 200, 2, 2] 0 Conv2D-13 [[1, 200, 2, 2]] [1, 394, 2, 2] 78,800 BatchNorm2D-13 [[1, 394, 2, 2]] [1, 394, 2, 2] 1,576 ReLU-13 [[1, 394, 2, 2]] [1, 394, 2, 2] 0 ConvBNLayer-13 [[1, 200, 2, 2]] [1, 394, 2, 2] 0 DepthwiseSeparable-6 [[1, 200, 4, 4]] [1, 394, 2, 2] 0 Conv2D-14 [[1, 394, 2, 2]] [1, 394, 2, 2] 3,546 BatchNorm2D-14 [[1, 394, 2, 2]] [1, 394, 2, 2] 1,576 ReLU-14 [[1, 394, 2, 2]] [1, 394, 2, 2] 0 ConvBNLayer-14 [[1, 394, 2, 2]] [1, 394, 2, 2] 0 Conv2D-15 [[1, 394, 2, 2]] [1, 445, 2, 2] 175,330 BatchNorm2D-15 [[1, 445, 2, 2]] [1, 445, 2, 2] 1,780 ReLU-15 [[1, 445, 2, 2]] [1, 445, 2, 2] 0 ConvBNLayer-15 [[1, 394, 2, 2]] [1, 445, 2, 2] 0 DepthwiseSeparable-7 [[1, 394, 2, 2]] [1, 445, 2, 2] 0 Conv2D-16 [[1, 445, 2, 2]] [1, 445, 2, 2] 4,005 BatchNorm2D-16 [[1, 445, 2, 2]] [1, 445, 2, 2] 1,780 ReLU-16 [[1, 445, 2, 2]] [1, 445, 2, 2] 0 ConvBNLayer-16 [[1, 445, 2, 2]] [1, 445, 2, 2] 0 Conv2D-17 [[1, 445, 2, 2]] [1, 512, 2, 2] 227,840 BatchNorm2D-17 [[1, 512, 2, 2]] [1, 512, 2, 2] 2,048 ReLU-17 [[1, 512, 2, 2]] [1, 512, 2, 2] 0 ConvBNLayer-17 [[1, 445, 2, 2]] [1, 512, 2, 2] 0 DepthwiseSeparable-8 [[1, 445, 2, 2]] [1, 512, 2, 2] 0 Conv2D-18 [[1, 512, 2, 2]] [1, 512, 2, 2] 4,608 BatchNorm2D-18 [[1, 512, 2, 2]] [1, 512, 2, 2] 2,048 ReLU-18 [[1, 512, 2, 2]] [1, 512, 2, 2] 0 ConvBNLayer-18 [[1, 512, 2, 2]] [1, 512, 2, 2] 0 Conv2D-19 [[1, 512, 2, 2]] [1, 455, 2, 2] 232,960 BatchNorm2D-19 [[1, 455, 2, 2]] [1, 455, 2, 2] 1,820 ReLU-19 [[1, 455, 2, 2]] [1, 455, 2, 2] 0 ConvBNLayer-19 [[1, 512, 2, 2]] [1, 455, 2, 2] 0 DepthwiseSeparable-9 [[1, 512, 2, 2]] [1, 455, 2, 2] 0 Conv2D-20 [[1, 455, 2, 2]] [1, 455, 2, 2] 4,095 BatchNorm2D-20 [[1, 455, 2, 2]] [1, 455, 2, 2] 1,820 ReLU-20 [[1, 455, 2, 2]] [1, 455, 2, 2] 0 ConvBNLayer-20 [[1, 455, 2, 2]] [1, 455, 2, 2] 0 Conv2D-21 [[1, 455, 2, 2]] [1, 414, 2, 2] 188,370 BatchNorm2D-21 [[1, 414, 2, 2]] [1, 414, 2, 2] 1,656 ReLU-21 [[1, 414, 2, 2]] [1, 414, 2, 2] 0 ConvBNLayer-21 [[1, 455, 2, 2]] [1, 414, 2, 2] 0 DepthwiseSeparable-10 [[1, 455, 2, 2]] [1, 414, 2, 2] 0 Conv2D-22 [[1, 414, 2, 2]] [1, 414, 2, 2] 3,726 BatchNorm2D-22 [[1, 414, 2, 2]] [1, 414, 2, 2] 1,656 ReLU-22 [[1, 414, 2, 2]] [1, 414, 2, 2] 0 ConvBNLayer-22 [[1, 414, 2, 2]] [1, 414, 2, 2] 0 Conv2D-23 [[1, 414, 2, 2]] [1, 324, 2, 2] 134,136 BatchNorm2D-23 [[1, 324, 2, 2]] [1, 324, 2, 2] 1,296 ReLU-23 [[1, 324, 2, 2]] [1, 324, 2, 2] 0 ConvBNLayer-23 [[1, 414, 2, 2]] [1, 324, 2, 2] 0 DepthwiseSeparable-11 [[1, 414, 2, 2]] [1, 324, 2, 2] 0 Conv2D-24 [[1, 324, 2, 2]] [1, 324, 1, 1] 2,916 BatchNorm2D-24 [[1, 324, 1, 1]] [1, 324, 1, 1] 1,296 ReLU-24 [[1, 324, 1, 1]] [1, 324, 1, 1] 0 ConvBNLayer-24 [[1, 324, 2, 2]] [1, 324, 1, 1] 0 Conv2D-25 [[1, 324, 1, 1]] [1, 383, 1, 1] 124,092 BatchNorm2D-25 [[1, 383, 1, 1]] [1, 383, 1, 1] 1,532 ReLU-25 [[1, 383, 1, 1]] [1, 383, 1, 1] 0 ConvBNLayer-25 [[1, 324, 1, 1]] [1, 383, 1, 1] 0 DepthwiseSeparable-12 [[1, 324, 2, 2]] [1, 383, 1, 1] 0 Conv2D-26 [[1, 383, 1, 1]] [1, 383, 1, 1] 3,447 BatchNorm2D-26 [[1, 383, 1, 1]] [1, 383, 1, 1] 1,532 ReLU-26 [[1, 383, 1, 1]] [1, 383, 1, 1] 0 ConvBNLayer-26 [[1, 383, 1, 1]] [1, 383, 1, 1] 0 Conv2D-27 [[1, 383, 1, 1]] [1, 1024, 1, 1] 392,192 BatchNorm2D-27 [[1, 1024, 1, 1]] [1, 1024, 1, 1] 4,096 ReLU-27 [[1, 1024, 1, 1]] [1, 1024, 1, 1] 0 ConvBNLayer-27 [[1, 383, 1, 1]] [1, 1024, 1, 1] 0 DepthwiseSeparable-13 [[1, 383, 1, 1]] [1, 1024, 1, 1] 0 AdaptiveAvgPool2D-1 [[1, 1024, 1, 1]] [1, 1024, 1, 1] 0 Linear-1 [[1, 1024]] [1, 1000] 1,025,000 ================================================================================= Total params: 2,700,966 Trainable params: 2,668,622 Non-trainable params: 32,344 --------------------------------------------------------------------------------- Input size (MB): 0.01 Forward/backward pass size (MB): 2.70 Params size (MB): 10.30 Estimated Total Size (MB): 13.01 ---------------------------------------------------------------------------------
{'total_params': 2700966, 'trainable_params': 2668622}
往届优秀学员作品展示
Paddle Lite和PaddleSlim的实践
- 项目背景
当我们在电脑端实现对输出模型的高精度保障之后,如何将其部署到移动设备,或者工业环境的嵌入式设备上,是一大难题。因为在落地应用中,模型的性能发挥可能受制于硬件设备的计算能力,传感器的精度,周围环境的噪声等等。因此,探讨如何将模型成功部署,显得尤为重要。
- 项目内容
选择适用的模型网络,先在Android设备上运行验证。再通过压缩进行对比实验。
- 实现方案
该项目使用MobileNetV3 Large的骨干网络,使用SSDLite结构,通过Paddle Lite将目标检测项目部署在Android上运行。然后进行模型的压缩和加速——使用PaddleSlim工具。 最终使用yolov3_mobilenet_v1_fruit模型检验在压缩前后模型的精度。
- 实现结果
模型压缩前:
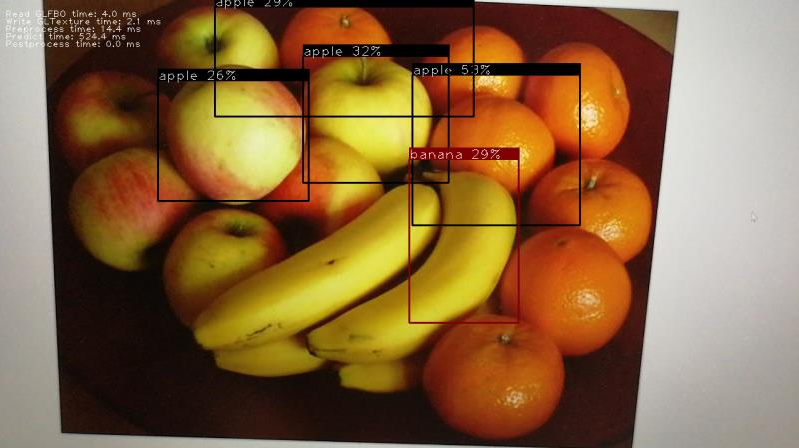
模型压缩后:
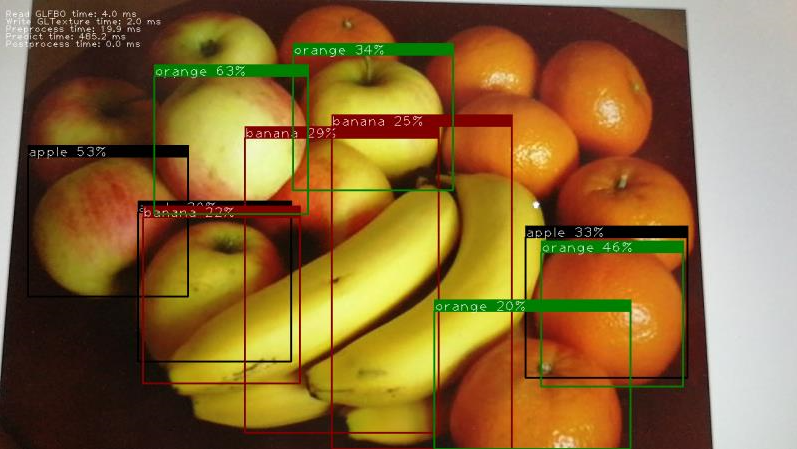
总结:裁剪后模型的大小变小了,从92.39M变成了75.71M 推理时间变短,从524.4ms变成了485.2ms 训练的评估结果裁剪前是mAP=68.79,裁剪后的mAP=67.52 两种训练方式都是按照相同的配置文件训练20000次
- 项目点评
完成了目标检测任务在移动端的部署,并且使用PaddleSlim对模型进行压缩对比精度、检测时间等指标,清晰完整的完成了作业的要求。
- 项目链接 https://aistudio.baidu.com/aistudio/projectdetail/518511